编辑推荐: |
本文来自于51cto,文章主要介绍如何导入图像并观察其属性、拆分图层以及查看灰度,希望对大家的学习能有所帮助。 |
|
本教程将介绍如何导入图像并观察其属性、拆分图层以及查看灰度。在正式开始之前,我们先来了解一些关于像素的基础知识。
计算机将图片以像素形式存储,这就像马赛克一样。如果像素太大,很难制作光滑的边缘和曲线。相反,我们使用的像素越多越小,看起来就会越平滑,或者说像素化程度越小,图像就会越好看,有时,这也被称为图像分辨率。
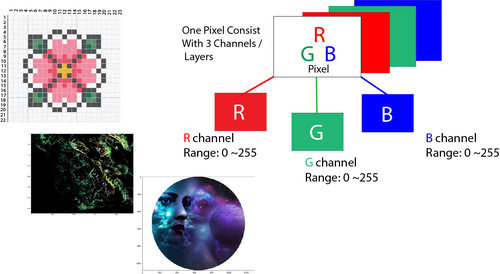
矢量图形是一种有点不同的存储图像方法,旨在避免与像素相关的问题。但是,即使是矢量图像,最终也会显示为像素一样的马赛克。颜色像素表示图像元素,描述每个像素的简单方法是使用三种颜色的组合,即红色,绿色,蓝色,这就是我们所说的RGB图像。
在RGB图像中,每个像素分别与红色,绿色,蓝色的值相关联的三个8比特数字表示。最后,如果使用放大镜观察缩放的图片,我们会看到图片由微小的光点或更具体的像素组成,更有趣的是这些小光点实际上具有多个不同颜色。
每张照片都以数字形式由像素组成,它们是构成图片的最小信息单位,通常是圆形或方形,它们通常布置在二维网格中。
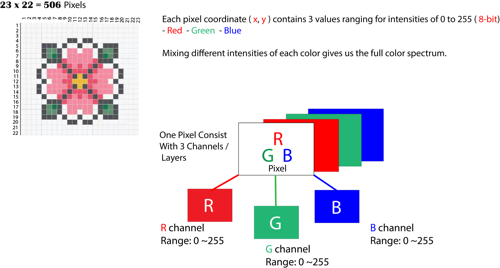
如果三个颜色都处于最大值,则意味着它们是255,那就会显示为白色,如果三种颜色都处于最小值,或者值为0,则颜色显示为黑色。反过来,这三者的组合将为我们提供特定的像素颜色。由于每个颜色数字都是8个比特,因此值范围为0-255。
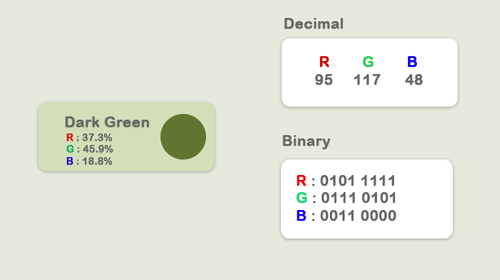
由于每个值可以具有256个不同的强度或亮度值,因此三种颜色总共有1680万个shade。
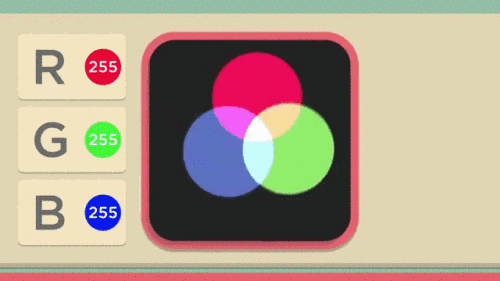
以下是Numpyand非常基本的图像数据分析步骤,其中一些涉及Python pacakges,如imageio,matplotlib等。
导入图像并观察其属性
拆分图层
Greyscale
对像素值使用逻辑运算符
使用逻辑运算符进行运算
卫星图像数据分析
导入图像
现在让我们加载图像并观察各种属性:
if __name__
== '__main__':
import imageio
import matplotlib.pyplot as plt
%matplotlib inline
pic = imageio.imread('F:/demo_2.jpg')
plt.figure(figsize = (15,15))
plt.imshow(pic)观察图像的基本属性
print('Type of the image : ' , type(pic))
print('Shape of the image : {}'.format(pic.shape))
print('Image Hight {}'.format(pic.shape[0]))
print('Image Width {}'.format(pic.shape[1]))
print('Dimension of Image {}'.format(pic.ndim))
Type of the image :
Shape of the image : (562, 960, 3)
Image Hight 562
Image Width 960
Dimension of Image 3 |
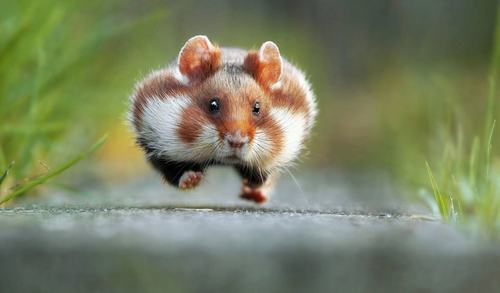
ndarray的形状表明它是一个三层矩阵,这里的前两个数字是长度和宽度,第三个数字(即3)是三层:Red,
Green, Blue。 因此,如果我们计算RGB图像的大小,则总大小将计为height x width
x 3
print('Image
size {}'.format(pic.size))
print('Maximum RGB value in this image {}'.format(pic.max()))
print('Minimum RGB value in this image {}'.format(pic.min()))
Image size 1618560
Maximum RGB value in this image 255
Minimum RGB value in this image 0 |
这些值对于验证很重要,因为8比特颜色强度不能超出0到255范围。
现在,使用图片分配变量,我们还可以访问图片的任何特定像素值,并进一步访问每个RGB通道。
'''
Let's pick a specific pixel located at 100 th
Rows and 50 th Column.
And view the RGB value gradually.
'''
pic[ 100, 50 ]
Image([109, 143, 46], dtype=uint8) |
在这种情况下:R = 109; G = 143; B = 46,我们可以意识到这个特殊像素中有很多绿色。现在,我们可以通过给出三个通道的索引值来特别选择其中一个数字:
0红色通道的索引值
1绿色通道的索引值
2蓝色通道的索引值
但是,在OpenCV中,图像不是RGB而是BGR,imageio.imread将图像加载为RGB(或RGBA),但OpenCV假定图像为BGR或BGRA(BGR是默认的OpenCV颜色格式)。
# A specific
pixel located at Row : 100 ; Column : 50
# Each channel's value of it, gradually R , G
, B
print('Value of only R channel {}'.format(pic[
100, 50, 0]))
print('Value of only G channel {}'.format(pic[
100, 50, 1]))
print('Value of only B channel {}'.format(pic[
100, 50, 2]))
Value of only R channel 109
Value of only G channel 143
Value of only B channel 46 |
好的,现在让我们快速查看整个图像中的每个频道。
plt.title('R
channel')
plt.ylabel('Height {}'.format(pic.shape[0]))
plt.xlabel('Width {}'.format(pic.shape[1]))
plt.imshow(pic[ : , : , 0])
plt.show() |
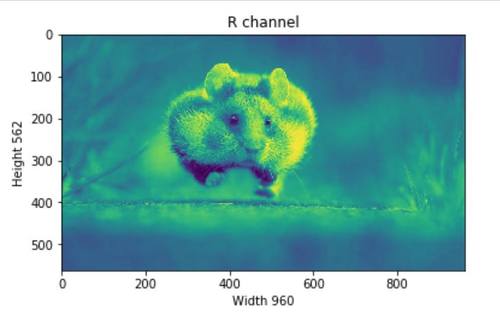
plt.title('G
channel')
plt.ylabel('Height {}'.format(pic.shape[0]))
plt.xlabel('Width {}'.format(pic.shape[1]))
plt.imshow(pic[ : , : , 1])
plt.show() |
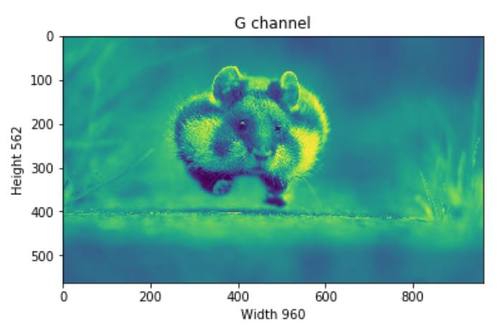
plt.title('B
channel')
plt.ylabel('Height {}'.format(pic.shape[0]))
plt.xlabel('Width {}'.format(pic.shape[1]))
plt.imshow(pic[ : , : , 2])
plt.show() |
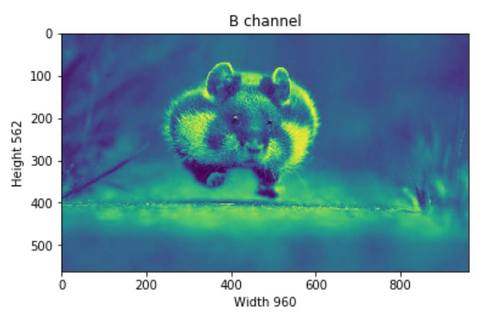
现在,我们可以更改RGB值的数量。例如,让我们对红色、绿色、蓝色图层设置跟随行值的强度。
R频道:行 - 100到110
G频道:行 - 200到210
B频道:行 - 300到310
我们将加载一次图像,以便可以同时显示每个层的变化。
pic = imageio.imread('F:/demo_2.jpg')
pic[50:150 , : , 0] = 255 # full intensity to
those pixel's R channel
plt.figure( figsize = (10,10))
plt.imshow(pic)
plt.show() |
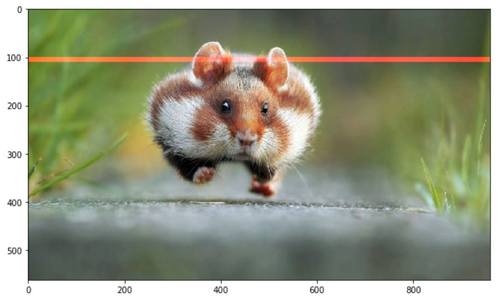
pic[200:300
, : , 1] = 255 # full intensity to those pixel's
G channel
plt.figure( figsize = (10,10))
plt.imshow(pic)
plt.show() |
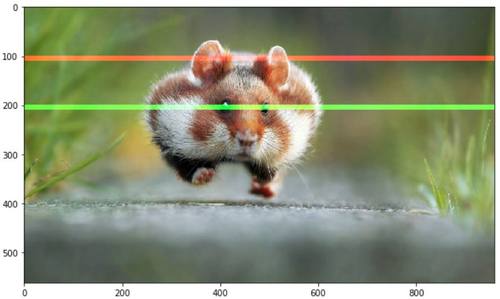
pic[350:450
, : , 2] = 255 # full intensity to those pixel's
B channel
plt.figure( figsize = (10,10))
plt.imshow(pic)
plt.show() |
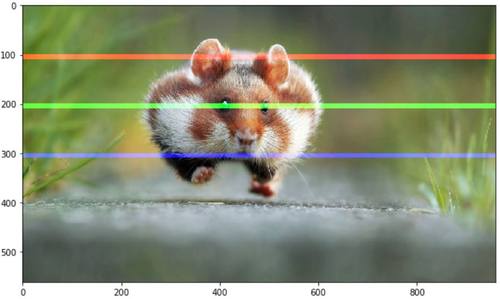
为了更清楚,让我们也改变列部分,这次我们将同时更改RGB通道。
# set value
200 of all channels to those pixels which turns
them to white
pic[ 50:450 , 400:600 , [0,1,2] ] = 200
plt.figure( figsize = (10,10))
plt.imshow(pic)
plt.show() |
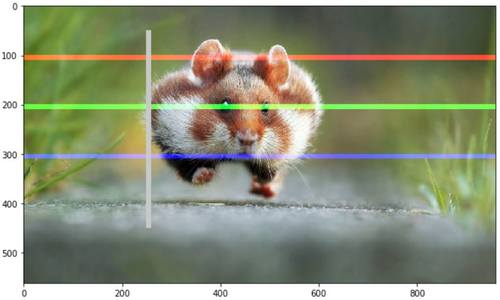
拆分图层
现在,我们知道图像的每个像素都由三个整数表示,将图像分割成单独的颜色分片只需拉出图像阵列的正确切片。
import numpy
as np
pic = imageio.imread('F:/demo_2.jpg')
fig, ax = plt.subplots(nrows = 1, ncols=3, figsize=(15,5))
for c, ax in zip(range(3), ax):
# create zero matrix
split_img = np.zeros(pic.shape, dtype="uint8")
# 'dtype' by default: 'numpy.float64'
# assing each channel
split_img[ :, :, c] = pic[ :, :, c]
# display each channel
ax.imshow(split_img) |
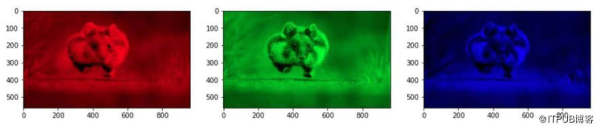
灰度
黑白图像存储在二维阵列中,有两种类型的黑白图像:
Greyscale:灰色阴影范围:0~255
Binary:像素为黑色或白色:0或255
现在,Greyscaling是一个将图像从全色转换为灰色阴影的过程。在图像处理工具中,例如:在OpenCV中,许多功能在处理之前使用灰度图像,这样做是因为它简化了图像,几乎可以降噪并增加处理时间,因为图像中的信息较少。
在python中有两种方法可以将图像转换为灰度,但使用matplotlib的简单方法是使用此公式获取原始图像的RGB值的加权平均值。
Y' = 0.299 R
+ 0.587 G + 0.114 B
pic = imageio.imread('F:/demo_2.jpg')
gray = lambda rgb : np.dot(rgb[... , :3] , [0.299
, 0.587, 0.114])
gray = gray(pic)
plt.figure( figsize = (10,10))
plt.imshow(gray, cmap = plt.get_cmap(name = 'gray'))
plt.show() |
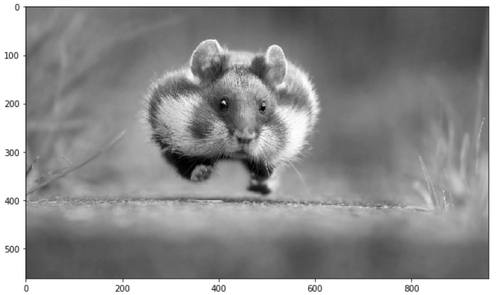
然而,GIMP将颜色转换为灰度图像软件有三种算法来完成任务。
灰度的Lightness 等级计算为
Lightness =
? × (max(R,G,B) + min(R,G,B)) |
灰度的Luminosity 等级计算为
Luminosity =
0.21 × R + 0.72 × G + 0.07 × B |
灰度的Average 计算为
Average Brightness
= (R + G + B) ÷ 3 |
让我们尝试一下算法,Luminosity效果如何?
pic = imageio.imread('F:/demo_2.jpg')
gray = lambda rgb : np.dot(rgb[... , :3] , [0.21
, 0.72, 0.07])
gray = gray(pic)
plt.figure( figsize = (10,10))
plt.imshow(gray, cmap = plt.get_cmap(name = 'gray'))
plt.show()
'''
Let's take a quick overview some the changed properties
now the color image.
Like we observe some properties of color image,
same statements are applying
now for gray scaled image.
'''
print('Type of the image : ' , type(gray))
print()
print('Shape of the image : {}'.format(gray.shape))
print('Image Hight {}'.format(gray.shape[0]))
print('Image Width {}'.format(gray.shape[1]))
print('Dimension of Image {}'.format(gray.ndim))
print()
print('Image size {}'.format(gray.size))
print('Maximum RGB value in this image {}'.format(gray.max()))
print('Minimum RGB value in this image {}'.format(gray.min()))
print('Random indexes [X,Y] : {}'.format(gray[100,
50]))
Type of the image :
Shape of the image : (562,960)
Image Height 562
Image Widht 960
Dimension of Image 2
Image size 539520
Maximum RGB value in this image 254.9999999997
Minimum RGB value in this image 0.0
Random indexes [X,Y] : 129.07 |
使用逻辑运算符处理像素值
我们可以使用逻辑运算符创建相同大小的bullion ndarray。但是,这不会创建任何新数组,它只是将值返回到其主变量。例如,如果考虑在RGB图像中滤除一些低值像素或高值或(任何条件),可以先将RGB转换为灰度。
首先加载图像并在屏幕上显示:
pic = imageio.imread('F:/demo_1.jpg')
plt.figure(figsize = (10,10))
plt.imshow(pic)
plt.show() |
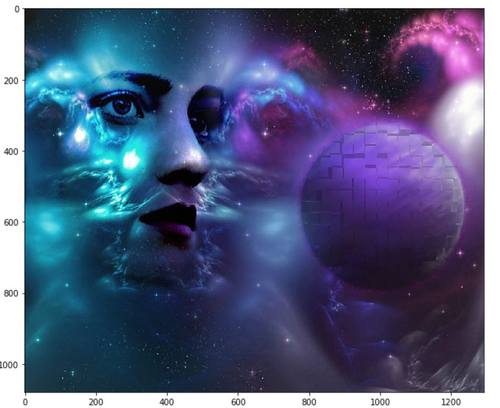
接下来,我们考虑转储该图像,比如我们想要过滤所有低于20 的像素值。为此,我们将使用逻辑运算符执行此任务,返回所有索引的True值。
low_pixel =
pic < 20
# to ensure of it let's check if all values in
low_pixel are True or not
if low_pixel.any() == True:
print(low_pixel.shape)
(1079, 1293, 3) |
正如上文所说,传统上不使用宿主变量,但我之所以提到是因为它只保留True值。 所以,如果我们看到low_pixel和pic的
shape,我们会发现它们都具有相同的 shape。
print(pic.shape)
print(low_pixel.shape)
(1079, 1293, 3)
(1079, 1293, 3) |
我们使用全局比较运算符为所有小于200的值生成低值滤波器。但是,我们可以使用此low_pixel数组作为索引将这些低值设置为某些特定值,这些值可能高于或低于先前的像素值。
# randomly choose
a value
import random
# load the orginal image
pic = imageio.imread('F:/demo_1.jpg')
# set value randomly range from 25 to 225 - these
value also randomly choosen
pic[low_pixel] = random.randint(25,225)
# display the image
plt.figure( figsize = (10,10))
plt.imshow(pic)
plt.show() |
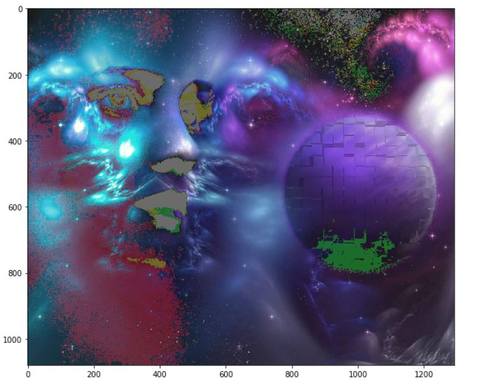
图层蒙版
图像蒙版是一种图像处理技术,用于去除具有模糊边缘,透明或头发部分的照片背景。
现在,我们将创建一个圆盘形状的蒙版。首先,我们将测量从图像中心到每个边界像素值的距离。我们设置一个比较方便的半径值,然后使用逻辑运算符创建一个圆盘,以下为代码:
if __name__
== '__main__':
# load the image
pic = imageio.imread('F:/demo_1.jpg')
# seperate the row and column values
total_row , total_col , layers = pic.shape
'''
Create vector.
Ogrid is a compact method of creating a multidimensional-
ndarray operations in single lines.
for ex:
>>> ogrid[0:5,0:5]
output: [array([[0],
[1],
[2],
[3],
[4]]),
array([[0, 1, 2, 3, 4]])]
'''
x , y = np.ogrid[:total_row , :total_col]
# get the center values of the image
cen_x , cen_y = total_row/2 , total_col/2
'''
Measure distance value from center to each border
pixel.
To make it easy, we can think it's like, we draw
a line from center-
to each edge pixel value --> s**2 = (Y-y)**2
+ (X-x)**2
'''
distance_from_the_center = np.sqrt((x-cen_x)**2
+ (y-cen_y)**2)
# Select convenient radius value
radius = (total_row/2)
# Using logical operator '>'
'''
logical operator to do this task which will return
as a value
of True for all the index according to the given
condition
'''
circular_pic = distance_from_the_center > radius
'''
let assign value zero for all pixel value that
outside the cirular disc.
All the pixel value outside the circular disc,
will be black now.
'''
pic[circular_pic] = 0
plt.figure(figsize = (10,10))
plt.imshow(pic)
plt.show() |
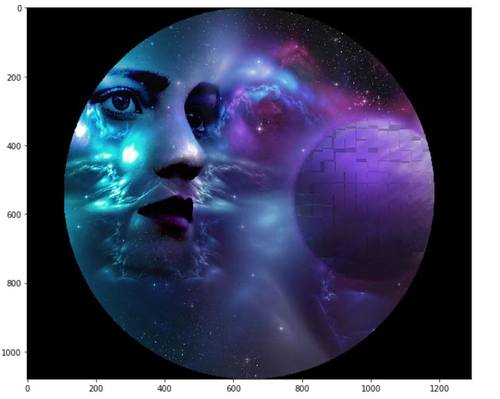
卫星图像处理
卫星图像及其处理系统非常有用,我们可以用于做一些分析任务。
# load the image
pic = imageio.imread('F:\satimg.jpg')
plt.figure(figsize = (10,10))
plt.imshow(pic)
plt.show() |
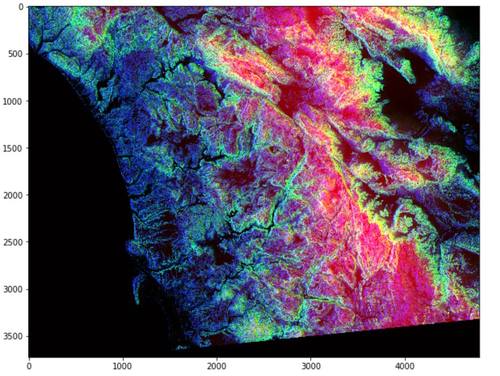
我们来看一些基本信息:
print(f'Shape
of the image {pic.shape}')
print(f'hieght {pic.shape[0]} pixels')
print(f'width {pic.shape[1]} pixels')
Shape of the image (3725, 4797, 3)
hieght 3725 pixels
width 4797 pixels |
这张图片上有一些有趣的东西,像许多其他的图像可视化一样,每个RGB层中的颜色都有自己的意思。例如,红色的强度将表示像素中的地理数据点的高度,蓝色的强度表示方位的度量,绿色表示斜率。这些颜色将有助于以更快,更有效的方式传达此信息,而不是显示数字。
红色像素表示:Altitude·
蓝色像素表示:Aspect
绿色像素表示: Slope
通过观察彩色图像,我们可以分辨出海拔是多少,斜率是多少,以及Slope是什么,这就是为颜色加载更多含义以表示更科学的分析的想法。
检测每个通道的像素
# Only Red Pixel
value , higher than 180
pic = imageio.imread('F:\satimg.jpg')
red_mask = pic[:, :, 0] < 180
pic[red_mask] = 0
plt.figure(figsize=(15,15))
plt.imshow(pic)
# Only Green Pixel value , higher than 180
pic = imageio.imread('F:\satimg.jpg')
green_mask = pic[:, :, 1] < 180
pic[green_mask] = 0
plt.figure(figsize=(15,15))
plt.imshow(pic)
# Only Blue Pixel value , higher than 180
pic = imageio.imread('F:\satimg.jpg')
blue_mask = pic[:, :, 2] < 180
pic[blue_mask] = 0
plt.figure(figsize=(15,15))
plt.imshow(pic)
# Composite mask using logical_and
pic = imageio.imread('F:\satimg.jpg')
final_mask = np.logical_and(red_mask, green_mask,
blue_mask)
pic[final_mask] = 40
plt.figure(figsize=(15,15))
plt.imshow(pic) |
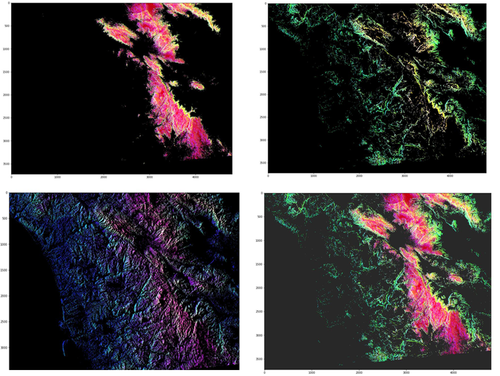
|