编辑推荐: |
本文来自云社区,本文通过使用Spark
Machine Learning Library和PySpark来解决一个文本多分类问题。 |
|
【导读】我们知道,Apache Spark在处理实时数据方面的能力非常出色,目前也在工业界广泛使用。本文通过使用Spark
Machine Learning Library和PySpark来解决一个文本多分类问题,内容包括:数据提取、Model
Pipeline、训练/测试数据集划分、模型训练和评价等,具体细节可以参考下面全文。
Multi-Class Text Classification with PySpark
Apache Spark受到越来越多的关注,主要是因为它处理实时数据的能力。每天都有大量的数据需要被处理,如何实时地分析这些数据变得极其重要。另外,Apache
Spark可以再不采样的情况下快速处理大量的数据。许多工业界的专家提供了理由: why you should
use Spark for Machine Learning?
数据
我们的任务,是将旧金山犯罪记录(San Francisco Crime
Description)分类到33个类目中。
给定一个犯罪描述,我们想知道它属于33类犯罪中的哪一类。分类器假设每个犯罪一定属于且仅属于33类中的一类。这是一个多分类的问题。
输入:犯罪描述。例如:“ STOLEN AUTOMOBILE”
输出:类别。例如:VEHICLE THEFT
为了解决这个问题,我们在Spark的有监督学习算法中用了一些特征提取技术。
数据提取
利用Spark的csv库直接载入CSV格式的数据:
from pyspark.sql
import SQLContext
from pyspark import SparkContext
sc =SparkContext()
sqlContext = SQLContext(sc)
data = sqlContext.read.format('com.databricks.spark.csv') .options(header='true',
inferschema='true').load('train.csv') |
除去一些不要的列,并展示前五行:
drop_list = ['Dates',
'DayOfWeek', 'PdDistrict', 'Resolution', 'Address',
'X', 'Y']
data = data.select([column for column in data.columns
if column not in drop_list])
data.show(5) |
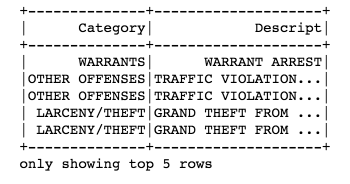
利用printSchema()方法来显示数据的结构:

包含数量最多的20类犯罪:
from pyspark.sql.functions
import col
data.groupBy("Category") \
.count() \
.orderBy(col("count").desc()) \
.show() |
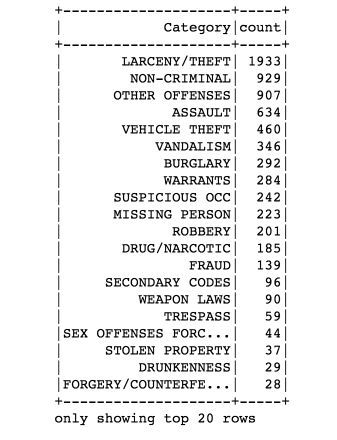
包含犯罪数量最多的20个描述:
from pyspark.sql.functions
import col
data.groupBy("Category") \
.count() \
.orderBy(col("count").desc()) \
.show() |
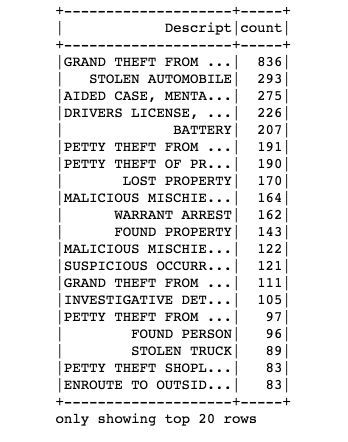
流水线(Model Pipeline)
我们的流程和scikit-learn版本的很相似,包含3个步骤:
1. regexTokenizer:利用正则切分单词
2. stopwordsRemover:移除停用词
3. countVectors:构建词频向量
from pyspark.ml.feature
import RegexTokenizer, StopWordsRemover, CountVectorizer
from pyspark.ml.classification import LogisticRegression
# regular expression tokenizer
regexTokenizer = RegexTokenizer(inputCol="Descript",
outputCol="words", pattern="\\W")
# stop words
add_stopwords = ["http","https","amp","rt","t","c","the"]
stopwordsRemover = StopWordsRemover(inputCol="words",
outputCol="filtered").
setStopWords(add_stopwords)
# bag of words count
countVectors = CountVectorizer(inputCol="filtered",
outputCol="features",
vocabSize=10000, minDF=5) |
StringIndexer
StringIndexer将一列字符串label编码为一列索引号(从0到label种类数-1),根据label出现的频率排序,最频繁出现的label的index为0。
在该例子中,label会被编码成从0到32的整数,最频繁的 label(LARCENY/THEFT)
会被编码成0。
from pyspark.ml
import Pipeline
from pyspark.ml.feature import OneHotEncoder,
StringIndexer, VectorAssembler
label_stringIdx = StringIndexer(inputCol = "Category",
outputCol = "label")
pipeline = Pipeline(stages=[regexTokenizer, stopwordsRemover,
countVectors,
label_stringIdx])
# Fit the pipeline to training documents.
pipelineFit = pipeline.fit(data)
dataset = pipelineFit.transform(data)
dataset.show(5) |

训练/测试数据集划分
# set seed for
reproducibility
(trainingData, testData) = dataset.randomSplit([0.7,
0.3], seed = 100)
print("Training Dataset Count: " + str(trainingData.count()))
print("Test Dataset Count: " + str(testData.count()))
|
训练数据量:5185
测试数据量:2104
模型训练和评价
1.以词频作为特征,利用逻辑回归进行分类
我们的模型在测试集上预测和打分,查看10个预测概率值最高的结果:
lr = LogisticRegression(maxIter=20,
regParam=0.3, elasticNetParam=0)
lrModel = lr.fit(trainingData)
predictions = lrModel.transform(testData)
predictions.filter(predictions['prediction']
== 0) \
.select("Descript","Category","probability","label" ,"prediction") \
.orderBy("probability", ascending=False)
\
.show(n = 10, truncate = 30) |
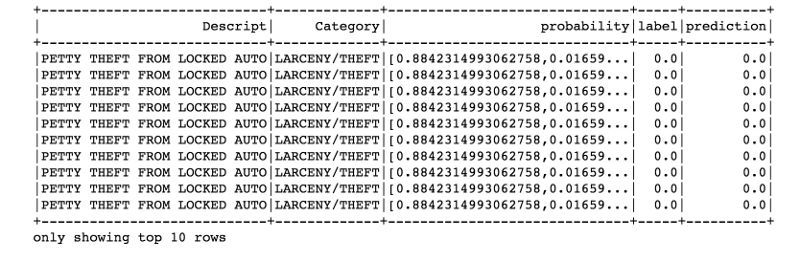
from pyspark.ml.evaluation
import MulticlassClassificationEvaluator
evaluator = MulticlassClassificationEvaluator (prediction Col="prediction")
evaluator.evaluate(predictions) |
准确率是0.9610787444388802,非常不错!
2.以TF-IDF作为特征,利用逻辑回归进行分类
from pyspark.ml.feature
import HashingTF, IDF
hashingTF = HashingTF(inputCol="filtered",
outputCol="rawFeatures", numFeatures=10000)
idf = IDF(inputCol="rawFeatures",
outputCol="features", minDocFreq=5)
#minDocFreq: remove sparse terms
pipeline = Pipeline(stages=[regexTokenizer,
stopwordsRemover, hashingTF, idf,
label_stringIdx])
pipelineFit = pipeline.fit(data)
dataset = pipelineFit.transform(data)
(trainingData, testData) = dataset.randomSplit ([0.7, 0.3], seed = 100)
lr = LogisticRegression(maxIter=20, regParam=0.3,
elasticNetParam=0)
lrModel = lr.fit(trainingData)
predictions = lrModel.transform(testData)
predictions.filter(predictions['prediction']
== 0) \
.select("Descript","Category","probability","label", "prediction") \
.orderBy("probability", ascending=False)
\
.show(n = 10, truncate = 30) |
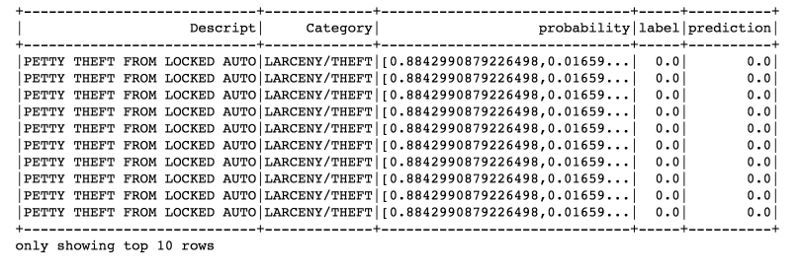
evaluator
= MulticlassClassificationEvaluator(predictionCol ="prediction")
evaluator.evaluate(predictions) |
准确率是0.9616202660247297,和上面结果差不多。
3.交叉验证
用交叉验证来优化参数,这里我们针对基于词频特征的逻辑回归模型进行优化。
pipeline = Pipeline(stages=[regexTokenizer,
stopwordsRemover, countVectors, label_stringIdx])
pipelineFit = pipeline.fit(data)
dataset = pipelineFit.transform(data)
(trainingData, testData) = dataset.randomSplit([0.7,
0.3], seed = 100)
lr = LogisticRegression(maxIter=20, regParam=0.3,
elasticNetParam=0)
from pyspark.ml.tuning import ParamGridBuilder,
CrossValidator
# Create ParamGrid for Cross Validation
paramGrid = (ParamGridBuilder()
.addGrid(lr.regParam, [0.1, 0.3, 0.5]) # regularization
parameter
.addGrid(lr.elasticNetParam, [0.0, 0.1, 0.2])
# Elastic Net Parameter (Ridge = 0)
# .addGrid(model.maxIter, [10, 20, 50]) #Number
of iterations
# .addGrid(idf.numFeatures, [10, 100, 1000]) #
Number of features
.build())
# Create 5-fold CrossValidator
cv = CrossValidator(estimator=lr, \
estimatorParamMaps=paramGrid, \
evaluator=evaluator, \
numFolds=5)
cvModel = cv.fit(trainingData)
predictions = cvModel.transform(testData)
# Evaluate best model
evaluator = MulticlassClassificationEvaluator(predictionCol= "prediction")
evaluator.evaluate(predictions) |
准确率变成了0.9851796929217101,获得了提升。
3.朴素贝叶斯
from pyspark.ml.classification
import NaiveBayes
nb = NaiveBayes(smoothing=1)
model = nb.fit(trainingData)
predictions = model.transform(testData)
predictions.filter(predictions['prediction']
== 0) \
.select("Descript","Category","probability","label", "prediction") \
.orderBy("probability", ascending=False)
\
.show(n = 10, truncate = 30) |
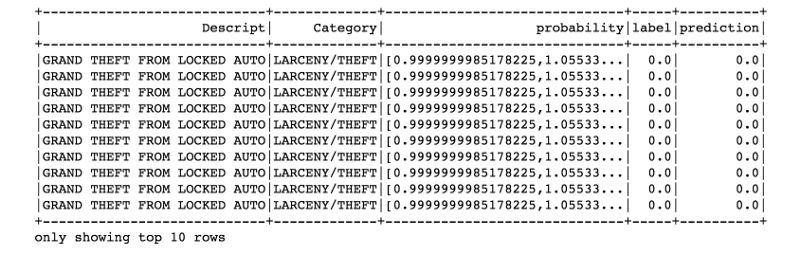
evaluator
= MulticlassClassificationEvaluator(predictionCol= "prediction")
evaluator.evaluate(predictions) |
准确率:0.9625414629888848
4.随机森林
from pyspark.ml.classification
import RandomForest Classifier
rf = RandomForestClassifier(labelCol="label",
\
featuresCol="features", \
numTrees = 100, \
maxDepth = 4, \
maxBins = 32)
# Train model with Training Data
rfModel = rf.fit(trainingData)
predictions = rfModel.transform(testData)
predictions.filter(predictions['prediction']
== 0) \
.select("Descript","Category","probability","label", "prediction") \
.orderBy("probability", ascending=False)
\
.show(n = 10, truncate = 30) |
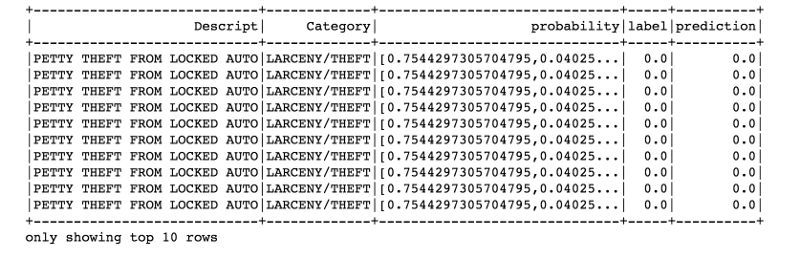
evaluator
= MulticlassClassificationEvaluator(predictionCol ="prediction")
evaluator.evaluate(predictions) |
准确率:0.6600326922344301
上面结果可以看出:随机森林是优秀的、鲁棒的通用的模型,但是对于高维稀疏数据来说,它并不是一个很好的选择。
明显,我们会选择使用了交叉验证的逻辑回归。
|