编辑推荐: |
本文主要介绍如何使用一个TensorFlow的可视化工具进行可视化神经网络,希望对您的学习有所帮助。
本文来自于莫烦PYTHON,由火龙果软件Alice编辑、推荐。 |
|
学会用 Tensorflow 自带的 tensorboard 去可视化我们所建造出来的神经网络是一个很好的学习理解方式.
用最直观的流程图告诉你你的神经网络是长怎样,有助于你发现编程中间的问题和疑问.
效果
好,我们开始吧。
这次我们会介绍如何可视化神经网络。因为很多时候我们都是做好了一个神经网络,但是没有一个图像可以展示给大家看。这一节会介绍一个TensorFlow的可视化工具
— tensorboard :) 通过使用这个工具我们可以很直观的看到整个神经网络的结构、框架。 以前几节的代码为例:相关代码
通过tensorflow的工具大致可以看到,今天要显示的神经网络差不多是这样子的
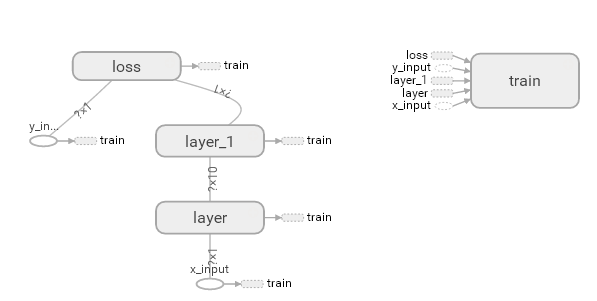
同时我们也可以展开看每个layer中的一些具体的结构:
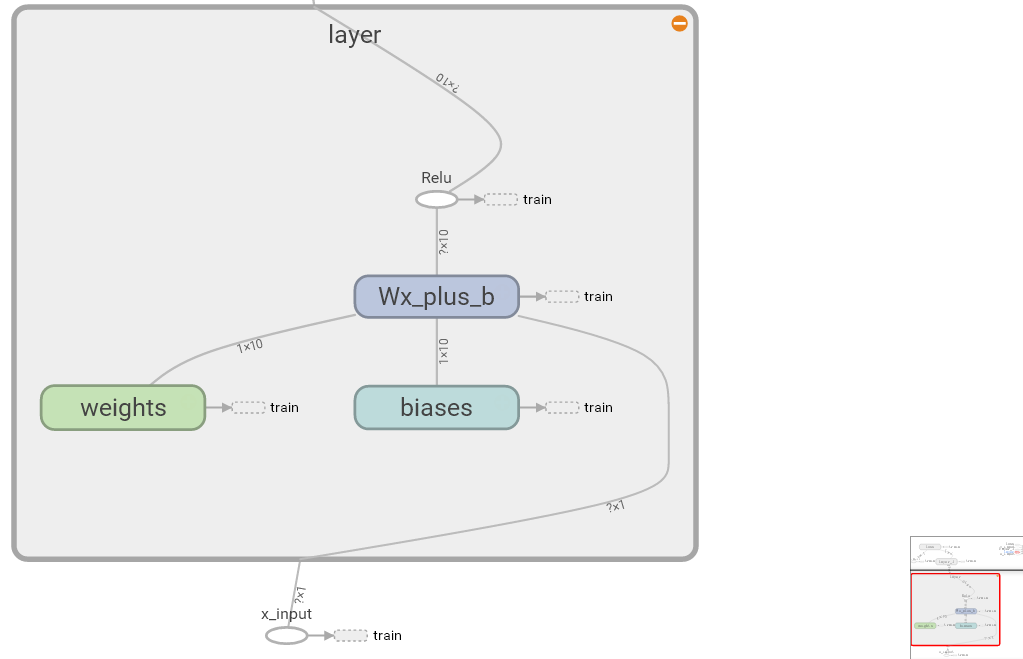
好,通过阅读代码和之前的图片我们大概知道了此处是有一个输入层(inputs),一个隐含层(layer),还有一个输出层(output)
现在可以看看如何进行可视化.
搭建图纸
首先从 Input 开始:
# define placeholder
for inputs to network
xs = tf.placeholder(tf.float32, [None, 1])
ys = tf.placeholder(tf.float32, [None, 1]) |
对于input我们进行如下修改: 首先,可以为xs指定名称为x_in:
xs= tf.placeholder(tf.float32,
[None, 1],name='x_in') |
然后再次对ys指定名称y_in:
ys= tf.placeholder(tf.loat32,
[None, 1],name='y_in') |
这里指定的名称将来会在可视化的图层inputs中显示出来
使用with tf.name_scope('inputs')可以将xs和ys包含进来,形成一个大的图层,图层的名字就是with
tf.name_scope()方法里的参数。
with tf.name_scope('inputs'):
# define placeholder for inputs to network
xs = tf.placeholder(tf.float32, [None, 1])
ys = tf.placeholder(tf.float32, [None, 1]) |
接下来开始编辑layer , 请看编辑前的程序片段 :
def add_layer(inputs,
in_size, out_size, activation_function=None):
# add one more layer and return the output of
this layer
Weights = tf.Variable(tf.random_normal([in_size,
out_size]))
biases = tf.Variable(tf.zeros([1, out_size]) +
0.1)
Wx_plus_b = tf.add(tf.matmul(inputs, Weights),
biases)
if activation_function is None:
outputs = Wx_plus_b
else:
outputs = activation_function(Wx_plus_b, )
return outputs |
这里的名字应该叫layer, 下面是编辑后的:
def add_layer(inputs,
in_size, out_size, activation_function=None):
# add one more layer and return the output of
this layer
with tf.name_scope('layer'):
Weights= tf.Variable(tf.random_normal([in_size,
out_size]))
# and so on... |
在定义完大的框架layer之后,同时也需要定义每一个’框架‘里面的小部件:(Weights biases
和 activation function): 现在现对 Weights 定义: 定义的方法同上,可以使用tf.name.scope()方法,同时也可以在Weights中指定名称W。
即为:
def add_layer(inputs,
in_size, out_size, activation_function=None):
#define layer name
with tf.name_scope('layer'):
#define weights name
with tf.name_scope('weights'):
Weights= tf.Variable(tf.random_normal([in_size,
out_size]),name='W')
#and so on...... |
接着继续定义biases , 定义方式同上。
def add_layer(inputs,
in_size, out_size, activation_function=None):
#define layer name
with tf.name_scope('layer'):
#define weights name
with tf.name_scope('weights')
Weights= tf.Variable(tf.random_normal([in_size,
out_size]),name='W')
# define biase
with tf.name_scope('Wx_plus_b'):
Wx_plus_b = tf.add(tf.matmul(inputs, Weights),
biases)
# and so on.... |
activation_function 的话,可以暂时忽略。因为当你自己选择用
tensorflow 中的激励函数(activation function)的时候,tensorflow会默认添加名称。
最终,layer形式如下:
def add_layer(inputs,
in_size, out_size, activation_function=None):
# add one more layer and return the output of
this layer
with tf.name_scope('layer'):
with tf.name_scope('weights'):
Weights = tf.Variable(
tf.random_normal([in_size, out_size]),
name='W')
with tf.name_scope('biases'):
biases = tf.Variable(
tf.zeros([1, out_size]) + 0.1,
name='b')
with tf.name_scope('Wx_plus_b'):
Wx_plus_b = tf.add(
tf.matmul(inputs, Weights),
biases)
if activation_function is None:
outputs = Wx_plus_b
else:
outputs = activation_function(Wx_plus_b, )
return outputs |
效果如下:(有没有看见刚才定义layer里面的“内部构件”呢?)
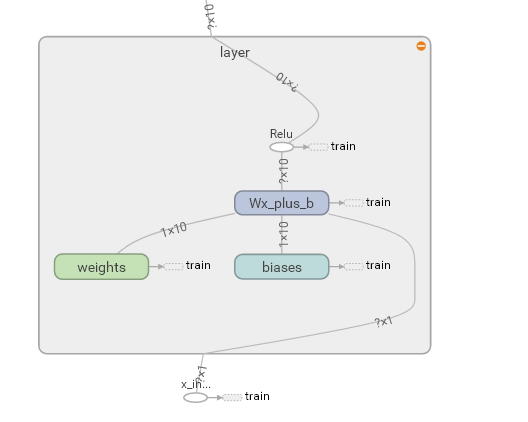
最后编辑loss部分:将with tf.name_scope()添加在loss上方,并为它起名为loss
# the error between
prediciton and real data
with tf.name_scope('loss'):
loss = tf.reduce_mean(
tf.reduce_sum(
tf.square(ys - prediction),
eduction_indices=[1]
)) |
这句话就是“绘制” loss了, 如下:
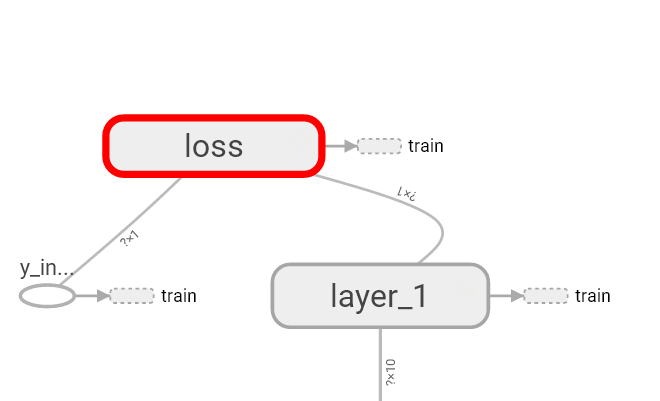
使用with tf.name_scope()再次对train_step部分进行编辑,如下:
with tf.name_scope('train'):
train_step = tf.train.GradientDescentOptimizer(0.1).minimize(loss) |
我们需要使用 tf.summary.FileWriter() (tf.train.SummaryWriter()
这种方式已经在 tf >= 0.12 版本中摒弃) 将上面‘绘画’出的图保存到一个目录中,以方便后期在浏览器中可以浏览。
这个方法中的第二个参数需要使用sess.graph , 因此我们需要把这句话放在获取session的后面。
这里的graph是将前面定义的框架信息收集起来,然后放在logs/目录下面。
sess = tf.Session()
# get session
# tf.train.SummaryWriter soon be deprecated, use
following
writer = tf.summary.FileWriter("logs/",
sess.graph) |
最后在你的terminal(终端)中 ,使用以下命令
tensorboard --logdir
logs |
同时将终端中输出的网址复制到浏览器中,便可以看到之前定义的视图框架了。
tensorboard 还有很多其他的参数,希望大家可以多多了解, 可以使用 tensorboard
--help 查看tensorboard的详细参数 最终的全部代码在这里
可能会遇到的问题
(1) 而且与 tensorboard 兼容的浏览器是 “Google Chrome”. 使用其他的浏览器不保证所有内容都能正常显示.
(2) 同时注意, 如果使用 http://0.0.0.0:6006 网址打不开的朋友们, 请使用
http://localhost:6006, 大多数朋友都是这个问题.
(3) 请确保你的 tensorboard 指令是在你的 logs
文件根目录执行的. 如果在其他目录下, 比如 Desktop 等, 可能不会成功看到图. 比如在下面这个目录,
你要 cd 到 project 这个地方执行 /project > tensorboard --logdir
logs
- project
- logs
model.py
env.py |
(4) 讨论区的朋友使用 anaconda 下的 python3.5 的虚拟环境, 如果你输入 tensorboard
的指令, 出现报错: "tensorboard" is not recognized
as an internal or external command...
解决方法的关键就是需要激活TensorFlow. 管理员模式打开 Anaconda Prompt,
输入 activate tensorflow, 接着按照上面的流程执行 tensorboard 指令. |