Bisecting k-means聚类算法,即二分k均值算法,它是k-means聚类算法的一个变体,主要是为了改进k-means算法随机选择初始质心的随机性造成聚类结果不确定性的问题,而Bisecting
k-means算法受随机选择初始质心的影响比较小。
首先,我们考虑在欧几里德空间中,衡量簇的质量通常使用如下度量:误差平方和(Sum
of the Squared Error,简称SSE),也就是要计算执行聚类分析后,对每个点都要计算一个误差值,即非质心点到最近的质心的距离。那么,既然每个非质心点都已经属于某个簇,也就是要计算每个非质心点到其所在簇的质心的距离,最后将这些距离值相加求和,作为SSE去评估一个聚类的质量如何。我们的最终目标是,使得最终的SSE能够最小,也就是一个最小化目标SSE的问题。在n维欧几里德空间,SSE形式化地定义,计算公式如下:

Bisecting k-means聚类算法的基本思想是,通过引入局部二分试验,每次试验都通过二分具有最大SSE值的一个簇,二分这个簇以后得到的2个子簇,选择2个子簇的总SSE最小的划分方法,这样能够保证每次二分得到的2个簇是比较优的(也可能是最优的),也就是这2个簇的划分可能是局部最优的,取决于试验的次数。
Bisecting k-means聚类算法的具体执行过程,描述如下所示:
1、初始时,将待聚类数据集D作为一个簇C0,即C={C0},输入参数为:二分试验次数m、k-means聚类的基本参数;
2、取C中具有最大SSE的簇Cp,进行二分试验m次:调用k-means聚类算法,取k=2,将Cp分为2个簇:Ci1、Ci2,一共得到m个二分结果集合B={B1,B2,…,Bm},其中,Bi={Ci1,Ci2},这里Ci1和Ci2为每一次二分试验得到的2个簇;
3、计算上一步二分结果集合B中,每一个划分方法得到的2个簇的总SSE值,选择具有最小总SSE的二分方法得到的结果:Bj={Cj1,Cj2},并将簇Cj1、Cj2加入到集合C,并将Cp从C中移除;
4、重复步骤2和3,直到得到k个簇,即集合C中有k个簇。
聚类算法实现
基于上面描述的聚类执行过程,使用Java实现Bisecting k-means聚类,代码如下所示:
@Override public void clustering() { // parse sample files final List<Point2D> allPoints = Lists.newArrayList(); FileUtils.read2DPointsFromFiles(allPoints, "[\t,;\\s]+", inputFiles);
// 从文件中读取二维坐标点,加入到集合allPoints中 final int bisectingK = 2; int bisectingIterations = 0; int maxInterations = 20; List<Point2D> points = allPoints; final Map<CenterPoint,
Set<ClusterPoint<Point2D>>> clusteringPoints = Maps.newConcurrentMap();
// 最终的聚类结果集合 while(clusteringPoints.size() <= k) { // 当得到k个簇,则算法终止 LOG.info("Start bisecting iterations: #" + (++bisectingIterations) + ",
bisectingK=" + bisectingK + ",maxMovingPointRate=" + maxMovingPointRate + ", maxInterations=" + maxInterations + ", parallism=" + parallism); // for k=bisectingK, execute k-means clustering // bisecting trials KMeansClustering bestBisectingKmeans = null; double minTotalSSE = Double.MAX_VALUE; for (int i = 0; i < m; i++) {
// 执行二分试验:调用k-means聚类算法,将输入的点集进行二分,得到2个簇,试验执行m次 final KMeansClustering kmeans = new KMeansClustering(bisectingK, maxMovingPointRate,
maxInterations, parallism); kmeans.initialize(points); // the clustering result should have 2 clusters kmeans.clustering(); double currentTotalSSE = computeTotalSSE(kmeans.getCenterPointSet(),
kmeans.getClusteringResult()); // 计算一次二分试验中总的SSE的值 if(bestBisectingKmeans == null) { bestBisectingKmeans = kmeans; minTotalSSE = currentTotalSSE; } else { if(currentTotalSSE < minTotalSSE) {
// 记录总SSE最小的二分聚类,通过kmeans保存二分结果 bestBisectingKmeans = kmeans; minTotalSSE = currentTotalSSE; } } LOG.info("Bisecting trial <<" + i + ">> : minTotalSSE=" + minTotalSSE + ",
currentTotalSSE=" + currentTotalSSE); } LOG.info("Best biscting: minTotalSSE=" + minTotalSSE); // merge cluster points for choosing cluster bisected again int id = generateNewClusterId(clusteringPoints.keySet());
// 每次执行k-means聚类,都多了一个簇,为多出的这个簇分配一个编号 Set<CenterPoint> bisectedCentroids = bestBisectingKmeans.getCenterPointSet();
// 二分得到的2个簇的质心的集合 merge(clusteringPoints, id, bisectedCentroids,
bestBisectingKmeans.getClusteringResult().getClusteredPoints());
// 将二分得到的2个簇的集合,合并加入到最终结果的集合中 if(clusteringPoints.size() == k) { // 已经得到k个簇,算法终止 break; } // compute cluster to be bisected ClusterInfo cluster = chooseClusterToBisect(clusteringPoints); // remove centroid from collected clusters map clusteringPoints.remove(cluster.centroidToBisect); LOG.info("Cluster to be bisected: " + cluster); points = Lists.newArrayList(); for(ClusterPoint<Point2D> cp : cluster.clusterPointsToBisect) { points.add(cp.getPoint()); } LOG.info("Finish bisecting iterations: #" + bisectingIterations + ",
clusterSize=" + clusteringPoints.size()); } // finally transform to result format Iterator<Entry<CenterPoint,
Set<ClusterPoint<Point2D>>>> iter = clusteringPoints.entrySet().iterator(); while(iter.hasNext()) { // 构造最终输出结果的数据结构 Entry<CenterPoint, Set<ClusterPoint<Point2D>>> entry = iter.next(); clusteredPoints.put(entry.getKey().getId(), entry.getValue()); centroidSet.add(entry.getKey()); } } |
上面,我们调用chooseClusterToBisect方法区实现对具有最大的SSE的簇进行二分,具体选择的实现过程,代码如下所示:
private ClusterInfo chooseClusterToBisect(Map<CenterPoint,
Set<ClusterPoint<Point2D>>> clusteringPoints) { double maxSSE = 0.0; int clusterIdWithMaxSSE = -1; CenterPoint centroidToBisect = null; Set<ClusterPoint<Point2D>> clusterToBisect = null; Iterator<Entry<CenterPoint,
Set<ClusterPoint<Point2D>>>> iter = clusteringPoints.entrySet().iterator(); while(iter.hasNext()) { Entry<CenterPoint, Set<ClusterPoint<Point2D>>> entry = iter.next(); CenterPoint centroid = entry.getKey(); Set<ClusterPoint<Point2D>> cpSet = entry.getValue(); double sse = computeSSE(centroid, cpSet); // 计算一个簇的SSE值 if(sse > maxSSE) { maxSSE = sse; clusterIdWithMaxSSE = centroid.getId(); centroidToBisect = centroid; clusterToBisect = cpSet; } } return new ClusterInfo(clusterIdWithMaxSSE, centroidToBisect, clusterToBisect, maxSSE);
// 将待分裂的簇的信息保存在ClusterInfo对象中 }
private double computeSSE(CenterPoint centroid,
Set<ClusterPoint<Point2D>> cpSet)
{
// 计算某个簇的SSE
double sse = 0.0;
for(ClusterPoint<Point2D> cp : cpSet) {
// update cluster id for ClusterPoint object
cp.setClusterId(centroid.getId());
double distance = MetricUtils.euclideanDistance(cp.getPoint(),
centroid);
sse += distance * distance;
}
return sse;
} |
在二分试验过程中,因为每次二分都生成2个新的簇,通过计算这2个簇的总SSE的值,通过迭代计算,找到一个局部最小的总SSE对应的2个簇的划分方法,然后将聚类生成的2簇加入到最终的簇集合中,总SSE的计算法方法在computeTotalSSE方法中,如下所示:
聚类效果对比
下面,我们对比一下,k-means算法与Bisecting k-means多次运行的聚类结果对比,如下图所示:
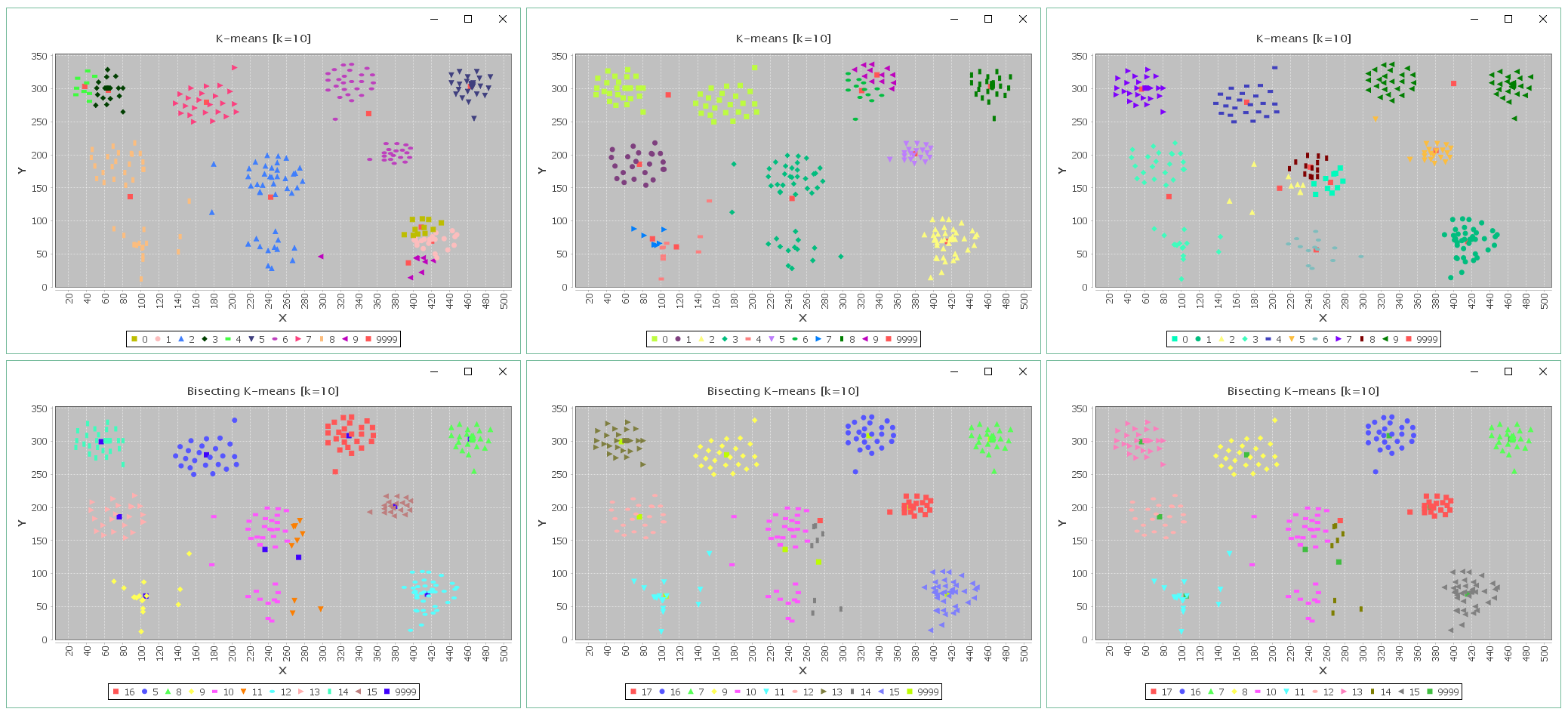
private double computeTotalSSE(Set<CenterPoint> centroids, ClusteringResult<Point2D> clusteringResult) { double sse = 0.0; for(CenterPoint center : centroids) { // 计算2个簇的总SSE值 int clusterId = center.getId(); for(ClusterPoint<Point2D> p : clusteringResult.getClusteredPoints().get(clusterId)) { double distance = MetricUtils.euclideanDistance(p.getPoint(), center); sse += distance * distance; } } return sse; } |
上图中,第一排是执行k-means聚类得到的效果图,第二排是执行Bisecting k-means聚类得到的效果图,其中编号为9999的点为质心点。第二排效果图看似多次计算质心没有变化,但是实际是变化的,只是质心点的位置比较稳定,例如,下面是两组质心点:
462.9642857142857,303.5,7 172.0,279.625,8 236.54285714285714,136.25714285714287,10 105.125,65.9375,11 75.91304347826087,185.7826086956522,12 56.03333333333333,299.53333333333336,13 273.5,117.5,14 415.5952380952381,68.71428571428571,15 329.04,308.68,16 374.35,200.55,17
172.0,279.625,5
462.9642857142857,303.5,8
105.125,65.9375,9
236.54285714285714,136.25714285714287,10
273.6666666666667,124.44444444444444,11
415.5952380952381,68.71428571428571,12
75.91304347826087,185.7826086956522,13
56.03333333333333,299.53333333333336,14
379.57894736842104,201.6315789473684,15
329.04,308.68,16 |
同k-means算法一样,Bisecting k-means算法不适用于非球形簇的聚类,而且不同尺寸和密度的类型的簇,也不太适合。 |