ÒÔ¾ö²ßÊ÷×÷Ϊ¿ªÊ¼£¬ÒòΪ¼òµ¥£¬¶øÇÒÒ²±È½ÏÈÝÒ×Óõ½£¬µ±Ç°µÄboosting»òrandom
forestÒ²Êdz£ÒÔÆäΪ»ù´¡µÄ
¾ö²ßÊ÷Ëã·¨±¾Éí²Î¿¼Ö®Ç°µÄblog£¬Æäʵ¾ÍÊÇ̰À·Ëã·¨£¬Ã¿´ÎÇзÖʹµÃÊý¾Ý±äµÃ×îΪÓÐÐò
ÄÇôÈçºÎÀ´¶¨ÒåÓÐÐò»òÎÞÐò£¿
ÎÞÐò£¬node impurity
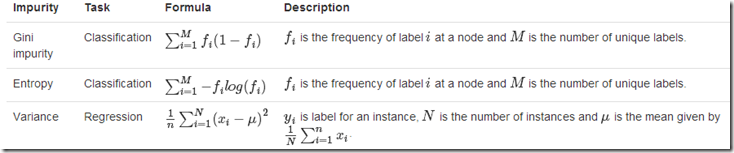
¶ÔÓÚ·ÖÀàÎÊÌ⣬ÎÒÃÇ¿ÉÒÔÓÃìØentropy»òGiniÀ´±íʾÐÅÏ¢µÄÎÞÐò³Ì¶È
¶ÔÓڻعéÎÊÌ⣬ÎÒÃÇÓ÷½²îVarianceÀ´±íʾÎÞÐò³Ì¶È£¬·½²îÔ½´ó£¬ËµÃ÷Êý¾Ý¼ä²îÒìÔ½´ó
information gain
ÓÃÓÚ±íʾ£¬Óɸ¸½Úµã»®·ÖºóµÃµ½×ӽڵ㣬Ëù´øÀ´µÄimpurityµÄϽµ£¬¼´ÓÐÐòÐÔµÄÔöÒæ

MLib¾ö²ßÊ÷µÄÀý×Ó
ÏÂÃæÖ±½Ó¿´¸öregressionµÄÀý×Ó£¬·ÖÀàµÄcase£¬²î²»¶à£¬
import org.apache.spark.mllib.tree.DecisionTree
import org.apache.spark.mllib.util.MLUtils
// Load and parse the data file.
// Cache the data since we will use it again to compute training error.
val data = MLUtils.loadLibSVMFile(sc, "data/mllib/sample_libsvm_data.txt").cache()
// Train a DecisionTree model.
// Empty categoricalFeaturesInfo indicates all features are continuous.
val categoricalFeaturesInfo = Map[Int, Int]()
val impurity = "variance"
val maxDepth = 5
val maxBins = 100
val model = DecisionTree.trainRegressor(data, categoricalFeaturesInfo, impurity,
maxDepth, maxBins)
// Evaluate model on training instances and compute training error
val labelsAndPredictions = data.map { point =>
val prediction = model.predict(point.features)
(point.label, prediction)
}
val trainMSE = labelsAndPredictions.map{ case(v, p) => math.pow((v - p), 2)}.mean()
println("Training Mean Squared Error = " + trainMSE)
println("Learned regression tree model:\n" + model) |
»¹ÊDZȽϼòµ¥µÄ£¬ÓÉÓÚÊǻع飬ËùÒÔimpurityµÄ¶¨ÒåΪvariance
maxDepth£¬×î´óÊ÷ÉÉèΪ5
maxBins£¬×î´óµÄ»®·ÖÊý
ÏÈÀí½âʲôÊÇbin£¬¾ö²ßÊ÷µÄËã·¨¾ÍÊǶÔfeatureµÄȡֵ²»¶ÏµÄ½øÐл®·Ö
¶ÔÓÚÀëÉ¢µÄfeature£¬±È½Ï¼òµ¥£¬Èç¹ûÓÐm¸öÖµ£¬×î¶à ¸ö»®·Ö£¬Èç¹ûÖµÊÇÓÐÐòµÄ£¬ÄÇô¾Í×î¶àm-1¸ö»®·Ö
±ÈÈçÄêÁäfeature£¬ÓÐÀÏ£¬ÖУ¬ÉÙ3¸öÖµ£¬Èç¹ûÎÞÐòÓÐ ¸ö£¬¼´3ÖÖ»®·Ö£¬ÀÏ|ÖУ¬ÉÙ£»ÀÏ£¬ÖÐ|ÉÙ£»ÀÏ£¬ÉÙ|ÖÐ
µ«Èç¹ûÊÇÓÐÐòµÄ£¬¼´°´ÀÏ£¬ÖУ¬ÉÙµÄÐò£¬ÄÇôֻÓÐm-1¸ö£¬¼´2ÖÖ»®·Ö£¬ÀÏ|ÖУ¬ÉÙ£»ÀÏ£¬ÖÐ|ÉÙ
¶ÔÓÚÁ¬ÐøµÄfeature£¬Æäʵ¾ÍÊǽøÐз¶Î§»®·Ö£¬¶ø»®·ÖµÄµã¾ÍÊÇsplit£¬»®·Ö³öµÄÇø¼ä¾ÍÊÇbin
¶ÔÓÚÁ¬Ðøfeature£¬ÀíÂÛÉÏ»®·ÖµãÊÇÎÞÊýµÄ£¬µ«ÊdzöÓÚЧÂÊÎÒÃÇ×ÜҪѡȡºÏÊʵĻ®·Öµã
Óиö±È½Ï³£Óõķ½·¨ÊÇÈ¡³öѵÁ·¼¯ÖиÃfeature³öÏÖ¹ýµÄÖµ×÷Ϊ»®·Öµã£¬
µ«¶ÔÓÚ·Ö²¼Ê½Êý¾Ý£¬È¡³öËùÓеÄÖµ½øÐÐÅÅÐòÒ²±È½Ï·Ñ×ÊÔ´£¬ËùÒÔ¿ÉÒÔ²ÉÈ¡sampleµÄ·½Ê½
Ô´Âë·ÖÎö
Ê×Ïȵ÷Óã¬DecisionTree.trainRegressor£¬ÀàËÆµ÷Óþ²Ì¬º¯Êý£¨object DecisionTree£©
org.apache.spark.mllib.tree.DecisionTree.scala
/**
* Method to train a decision tree model for regression.
*
* @param input Training dataset: RDD of [[org.apache.spark.mllib.regression.LabeledPoint]].
* Labels are real numbers.
* @param categoricalFeaturesInfo Map storing arity of categorical features.
* E.g., an entry (n -> k) indicates that feature n is categorical
* with k categories indexed from 0: {0, 1, ..., k-1}.
* @param impurity Criterion used for information gain calculation.
* Supported values: "variance".
* @param maxDepth Maximum depth of the tree.
* E.g., depth 0 means 1 leaf node; depth 1 means 1 internal node + 2 leaf nodes.
* (suggested value: 5)
* @param maxBins maximum number of bins used for splitting features
* (suggested value: 32)
* @return DecisionTreeModel that can be used for prediction
*/
def trainRegressor(
input: RDD[LabeledPoint],
categoricalFeaturesInfo: Map[Int, Int],
impurity: String,
maxDepth: Int,
maxBins: Int): DecisionTreeModel = {
val impurityType = Impurities.fromString(impurity)
train(input, Regression, impurityType, maxDepth, 0, maxBins, Sort, categoricalFeaturesInfo)
} |
µ÷Óþ²Ì¬º¯Êýtrain
def train(
input: RDD[LabeledPoint],
algo: Algo,
impurity: Impurity,
maxDepth: Int,
numClassesForClassification: Int,
maxBins: Int,
quantileCalculationStrategy: QuantileStrategy,
categoricalFeaturesInfo: Map[Int,Int]): DecisionTreeModel = {
val strategy = new Strategy(algo, impurity, maxDepth, numClassesForClassification, maxBins,
quantileCalculationStrategy, categoricalFeaturesInfo)
new DecisionTree(strategy).train(input)
} |
¿ÉÒÔ¿´µ½½«ËùÓвÎÊý·â×°µ½StrategyÀ࣬Ȼºó³õʼ»¯DecisionTreeÀà¶ÔÏ󣬼ÌÐøµ÷ÓóÉÔ±º¯Êýtrain
/**
* :: Experimental ::
* A class which implements a decision tree learning algorithm for classification and regression.
* It supports both continuous and categorical features.
* @param strategy The configuration parameters for the tree algorithm which specify the type
* of algorithm (classification, regression, etc.), feature type (continuous,
* categorical), depth of the tree, quantile calculation strategy, etc.
*/
@Experimental
class DecisionTree (private val strategy: Strategy) extends Serializable with Logging {
strategy.assertValid()
/**
* Method to train a decision tree model over an RDD
* @param input Training data: RDD of [[org.apache.spark.mllib.regression.LabeledPoint]]
* @return DecisionTreeModel that can be used for prediction
*/
def train(input: RDD[LabeledPoint]): DecisionTreeModel = {
// Note: random seed will not be used since numTrees = 1.
val rf = new RandomForest(strategy, numTrees = 1, featureSubsetStrategy = "all", seed = 0)
val rfModel = rf.train(input)
rfModel.trees(0)
}
} |
¿ÉÒÔ¿´µ½£¬ÕâÀïDecisionTreeµÄÉè¼ÆÊÇ»ùÓÚRandomForestµÄÌØÀý£¬¼´µ¥¿ÅÊ÷µÄRandomForest
ËùÒÔµ÷ÓÃRandomForest.train()£¬×îÖÕÒòΪֻÓÐÒ»¿ÃÊ÷£¬ËùÒÔÈ¡trees(0)
org.apache.spark.mllib.tree.RandomForest.scala
ÖØµã¿´Ï£¬RandomForestÀïÃæµÄtrain×öÁËʲô£¿
/**
* Method to train a decision tree model over an RDD
* @param input Training data: RDD of [[org.apache.spark.mllib.regression.LabeledPoint]]
* @return RandomForestModel that can be used for prediction
*/
def train(input: RDD[LabeledPoint]): RandomForestModel = {
//1. metadata
val retaggedInput = input.retag(classOf[LabeledPoint])
val metadata =
DecisionTreeMetadata.buildMetadata(retaggedInput, strategy, numTrees, featureSubsetStrategy)
// 2. Find the splits and the corresponding bins (interval between the splits) using a sample
// of the input data.
val (splits, bins) = DecisionTree.findSplitsBins(retaggedInput, metadata)
// 3. Bin feature values (TreePoint representation).
// Cache input RDD for speedup during multiple passes.
val treeInput = TreePoint.convertToTreeRDD(retaggedInput, bins, metadata)
val baggedInput = if (numTrees > 1) {
BaggedPoint.convertToBaggedRDD(treeInput, numTrees, seed)
} else {
BaggedPoint.convertToBaggedRDDWithoutSampling(treeInput)
}.persist(StorageLevel.MEMORY_AND_DISK)
// set maxDepth and compute memory usage
// depth of the decision tree
// Max memory usage for aggregates
// TODO: Calculate memory usage more precisely.
//........
/*
* The main idea here is to perform group-wise training of the decision tree nodes thus
* reducing the passes over the data from (# nodes) to (# nodes / maxNumberOfNodesPerGroup).
* Each data sample is handled by a particular node (or it reaches a leaf and is not used
* in lower levels).
*/
// FIFO queue of nodes to train: (treeIndex, node)
val nodeQueue = new mutable.Queue[(Int, Node)]()
val rng = new scala.util.Random()
rng.setSeed(seed)
// Allocate and queue root nodes.
val topNodes: Array[Node] = Array.fill[Node](numTrees)(Node.emptyNode(nodeIndex = 1))
Range(0, numTrees).foreach(treeIndex => nodeQueue.enqueue((treeIndex, topNodes(treeIndex))))
while (nodeQueue.nonEmpty) {
// Collect some nodes to split, and choose features for each node (if subsampling).
// Each group of nodes may come from one or multiple trees, and at multiple levels.
val (nodesForGroup, treeToNodeToIndexInfo) =
RandomForest.selectNodesToSplit(nodeQueue, maxMemoryUsage, metadata, rng)
// ¶Ôdecision treeûÓÐÒâÒ壬nodeQueueÖ»ÓÐÒ»¸önode£¬²»ÐèҪѡ
// 4. Choose node splits, and enqueue new nodes as needed.
DecisionTree.findBestSplits(baggedInput, metadata, topNodes, nodesForGroup,
treeToNodeToIndexInfo, splits, bins, nodeQueue, timer)
}
val trees = topNodes.map(topNode => new DecisionTreeModel(topNode, strategy.algo))
RandomForestModel.build(trees)
} |
1. DecisionTreeMetadata.buildMetadata
org.apache.spark.mllib.tree.impl.DecisionTreeMetadata.scala
ÕâÀïÉú³ÉһЩºóÃæÐèÒªÓõ½µÄmetadata
×î¹Ø¼üµÄÊǼÆËãÿ¸öfeatureµÄbinsºÍsplitsµÄÊýÄ¿£¬
¼ÆËãbinsµÄÊýÄ¿
//binsÊýÄ¿×î´ó²»Äܳ¬¹ýѵÁ·¼¯ÖÐÑù±¾µÄsize
val maxPossibleBins = math.min(strategy.maxBins, numExamples).toInt
//ÉèÖÃĬÈÏÖµ
val numBins = Array.fill[Int](numFeatures)(maxPossibleBins)
if (numClasses > 2) {
// Multiclass classification
val maxCategoriesForUnorderedFeature =
((math.log(maxPossibleBins / 2 + 1) / math.log(2.0)) + 1).floor.toInt
strategy.categoricalFeaturesInfo.foreach { case (featureIndex, numCategories) =>
// Decide if some categorical features should be treated as unordered features,
// which require 2 * ((1 << numCategories - 1) - 1) bins.
// We do this check with log values to prevent overflows in case numCategories is large.
// The next check is equivalent to: 2 * ((1 << numCategories - 1) - 1) <= maxBins
if (numCategories <= maxCategoriesForUnorderedFeature) {
unorderedFeatures.add(featureIndex)
numBins(featureIndex) = numUnorderedBins(numCategories)
} else {
numBins(featureIndex) = numCategories
}
}
} else {
// Binary classification or regression
strategy.categoricalFeaturesInfo.foreach { case (featureIndex, numCategories) =>
numBins(featureIndex) = numCategories
}
}
|
ÆäËûcase£¬binsÊýÄ¿µÈÓÚfeatureµÄnumCategories
¶ÔÓÚunorderedÇé¿ö£¬±È½ÏÌØÊ⣬
/** * Given the arity of a categorical feature (arity = number of categories), * return the number of bins for the feature if it is to be treated as an unordered feature. * There is 1 split for every partitioning of categories into 2 disjoint, non-empty sets; * there are math.pow(2, arity - 1) - 1 such splits. * Each split has 2 corresponding bins. */ def numUnorderedBins(arity: Int): Int = 2 * ((1 << arity - 1) - 1) |
¸ù¾ÝbinsÊýÄ¿£¬¼ÆËãsplits
/** * Number of splits for the given feature. * For unordered features, there are 2 bins per split. * For ordered features, there is 1 more bin than split. */ def numSplits(featureIndex: Int): Int = if (isUnordered(featureIndex)) { numBins(featureIndex) >> 1 } else { numBins(featureIndex) - 1 } |
2. DecisionTree.findSplitsBins
Ê×ÏÈÕÒ³öÿ¸öfeatureÉÏ¿ÉÄܳöÏÖµÄsplitsºÍÏàÓ¦µÄbins£¬ÕâÊǺóÐøËã·¨µÄ»ù´¡
ÕâÀïµÄ×¢ÊͽâÊÍÁËÉÏÃæÈçºÎ¼ÆËãsplitsºÍbinsÊýÄ¿µÄËã·¨
a£¬¶ÔÓÚÁ¬ÐøÊý¾Ý£¬¶ÔÓÚÒ»¸öfeature£¬splits = numBins
- 1£»ÉÏÃæÒ²ËµÁ˶ÔÓÚÁ¬ÐøÖµ£¬Æäʵsplits¿ÉÒÔÎÞÏ޵ģ¬ÈçºÎÕÒµ½numBins - 1¸ösplits£¬ºÜ¼òµ¥£¬ÕâÀïÓÃsample
b£¬¶ÔÓÚÀëÉ¢Êý¾Ý£¬Á½¸öcase
b.1, ÎÞÐòµÄfeature£¬ÓÃÓÚlow-arity£¨²ÎÊý½ÏÉÙ£©µÄmulticlass·ÖÀ࣬ÕâÖÖcaseÏ»®·ÖµÄ¿ÉÄÜÐԱȽ϶࣬image£¬ËùÒÔÓÃsubsets
of categoriesÀ´×÷Ϊ»®·Ö
b.2, ÓÐÐòµÄfeature£¬ÓÃÓÚregression£¬¶þÔª·ÖÀ࣬»òhigh-arityµÄ¶àÔª·ÖÀ࣬ÕâÖÖcaseÏ»®·ÖµÄ¿ÉÄܱȽÏÉÙ£¬m-1£¬ËùÒÔÓÃÿ¸öcategory×÷Ϊ»®·Ö
/**
* Returns splits and bins for decision tree calculation.
* Continuous and categorical features are handled differently.
*
* Continuous features:
* For each feature, there are numBins - 1 possible splits representing the possible binary
* decisions at each node in the tree.
* This finds locations (feature values) for splits using a subsample of the data.
*
* Categorical features:
* For each feature, there is 1 bin per split.
* Splits and bins are handled in 2 ways:
* (a) "unordered features"
* For multiclass classification with a low-arity feature
* (i.e., if isMulticlass && isSpaceSufficientForAllCategoricalSplits),
* the feature is split based on subsets of categories.
* (b) "ordered features"
* For regression and binary classification,
* and for multiclass classification with a high-arity feature,
* there is one bin per category.
*
* @param input Training data: RDD of [[org.apache.spark.mllib.regression.LabeledPoint]]
* @param metadata Learning and dataset metadata
* @return A tuple of (splits, bins).
* Splits is an Array of [[org.apache.spark.mllib.tree.model.Split]]
* of size (numFeatures, numSplits).
* Bins is an Array of [[org.apache.spark.mllib.tree.model.Bin]]
* of size (numFeatures, numBins).
*/
protected[tree] def findSplitsBins(
input: RDD[LabeledPoint],
metadata: DecisionTreeMetadata): (Array[Array[Split]], Array[Array[Bin]]) = {
val numFeatures = metadata.numFeatures
// Sample the input only if there are continuous features.
val hasContinuousFeatures = Range(0, numFeatures).exists(metadata.isContinuous)
val sampledInput = if (hasContinuousFeatures) { // ¶ÔÓÚÁ¬ÐøÌØÕ÷£¬È¡Öµ»á±È½Ï¶à£¬ÐèÒª×ö³éÑù
// Calculate the number of samples for approximate quantile calculation.
val requiredSamples = math.max(metadata.maxBins * metadata.maxBins, 10000) // ³éÑùÊýÒªÔ¶´óÓÚͰÊý
val fraction = if (requiredSamples < metadata.numExamples) { // ÉèÖóéÑù±ÈÀý
requiredSamples.toDouble / metadata.numExamples
} else {
1.0
}
input.sample(withReplacement = false, fraction, new XORShiftRandom().nextInt()).collect()
} else {
new Array[LabeledPoint](0)
}
metadata.quantileStrategy match {
case Sort =>
val splits = new Array[Array[Split]](numFeatures) // ³õʼ»¯splitsºÍbins
val bins = new Array[Array[Bin]](numFeatures)
// Find all splits.
// Iterate over all features.
var featureIndex = 0
while (featureIndex < numFeatures) { // ±éÀúËùÓеÄfeature
val numSplits = metadata.numSplits(featureIndex) // È¡³öÇ°ÃæËã³öµÄsplitsºÍbinsµÄÊýÄ¿
val numBins = metadata.numBins(featureIndex)
if (metadata.isContinuous(featureIndex)) { // ¶ÔÓÚÁ¬ÐøµÄfeature
val numSamples = sampledInput.length
splits(featureIndex) = new Array[Split](numSplits)
bins(featureIndex) = new Array[Bin](numBins)
val featureSamples = sampledInput.map(lp => lp.features(featureIndex)).sorted
// ´ÓsampledInputÀïÃæÈ¡³ö¸ÃfeatureµÄËùÓÐȡֵ£¬ÅÅÐò
val stride: Double = numSamples.toDouble / metadata.numBins(featureIndex)
// È¡ÑùÊý/ͰÊý£¬¾ö¶¨split(»®·Ö)µÄ²½³¤
logDebug("stride = " + stride)
for (splitIndex <- 0 until numSplits) { // ¿ªÊ¼»®·Ö
val sampleIndex = splitIndex * stride.toInt // »®·ÖÊý¡Á²½³¤£¬µÃµ½»®·ÖËùÓõÄsampleµÄindex
// Set threshold halfway in between 2 samples.
val threshold = (featureSamples(sampleIndex) + featureSamples(sampleIndex + 1)) / 2.0
// »®·ÖµãѡȡÔÚǰºóÁ½¸ösampleµÄ¾ùÖµ
splits(featureIndex)(splitIndex) =
new Split(featureIndex, threshold, Continuous, List()) // ´´½¨Split¶ÔÏó
}
bins(featureIndex)(0) = new Bin(new DummyLowSplit(featureIndex, Continuous),
// ³õʼ»¯µÚÒ»¸ösplit£¬DummyLowSplit£¬È¡ÖµÊÇDouble.MinValue
splits(featureIndex)(0), Continuous, Double.MinValue)
for (splitIndex <- 1 until numSplits) { // ´´½¨ËùÓеÄbins
bins(featureIndex)(splitIndex) =
new Bin(splits(featureIndex)(splitIndex - 1), splits(featureIndex)(splitIndex),
Continuous, Double.MinValue)
}
bins(featureIndex)(numSplits) = new Bin(splits(featureIndex)(numSplits - 1),
// ³õʼ»¯×îºóÒ»¸ösplit£¬DummyHighSplit£¬È¡ÖµÊÇDouble.MaxValue
new DummyHighSplit(featureIndex, Continuous), Continuous, Double.MinValue)
} else { // ¶ÔÓÚ·ÖÀàµÄfeature
// Categorical feature
val featureArity = metadata.featureArity(featureIndex) // ÀëÉ¢ÌØÕ÷ÖеÄȡֵ¸öÊý
if (metadata.isUnordered(featureIndex)) { // ÎÞÐòµÄÀëÉ¢ÌØÕ÷
// TODO: The second half of the bins are unused. Actually, we could just use
// splits and not build bins for unordered features. That should be part of
// a later PR since it will require changing other code (using splits instead
// of bins in a few places).
// Unordered features
// 2^(maxFeatureValue - 1) - 1 combinations
splits(featureIndex) = new Array[Split](numSplits)
bins(featureIndex) = new Array[Bin](numBins)
var splitIndex = 0
while (splitIndex < numSplits) {
val categories: List[Double] =
extractMultiClassCategories(splitIndex + 1, featureArity)
splits(featureIndex)(splitIndex) =
new Split(featureIndex, Double.MinValue, Categorical, categories)
bins(featureIndex)(splitIndex) = {
if (splitIndex == 0) {
new Bin(
new DummyCategoricalSplit(featureIndex, Categorical),
splits(featureIndex)(0),
Categorical,
Double.MinValue)
} else {
new Bin(
splits(featureIndex)(splitIndex - 1),
splits(featureIndex)(splitIndex),
Categorical,
Double.MinValue)
}
}
splitIndex += 1
}
} else { // ÓÐÐòµÄÀëÉ¢ÌØÕ÷£¬²»ÐèÒªÊÂÏÈË㣬ÒòΪsplits¾ÍµÈÓÚfeatureArity
// Ordered features
// Bins correspond to feature values, so we do not need to compute splits or bins
// beforehand. Splits are constructed as needed during training.
splits(featureIndex) = new Array[Split](0)
bins(featureIndex) = new Array[Bin](0)
}
}
featureIndex += 1
}
(splits, bins)
case MinMax =>
throw new UnsupportedOperationException("minmax not supported yet.")
case ApproxHist =>
throw new UnsupportedOperationException("approximate histogram not supported yet.")
}
} |
3. TreePointºÍBaggedPoint
TreePointÊÇLabeledPointµÄÄÚ²¿Êý¾Ý½á¹¹£¬ÕâÀïÐèÒª×öת»»£¬
private def labeledPointToTreePoint(
labeledPoint: LabeledPoint,
bins: Array[Array[Bin]],
featureArity: Array[Int],
isUnordered: Array[Boolean]): TreePoint = {
val numFeatures = labeledPoint.features.size
val arr = new Array[Int](numFeatures)
var featureIndex = 0
while (featureIndex < numFeatures) {
arr(featureIndex) = findBin(featureIndex, labeledPoint, featureArity(featureIndex),
isUnordered(featureIndex), bins)
featureIndex += 1
}
new TreePoint(labeledPoint.label, arr) //Ö»Êǽ«labeledPointÖеÄvalueÌæ»»³Éarr
} |
arrÊÇfindBinµÄ½á¹û£¬ ÕâÀïÖ÷ÒªÊÇÕë¶ÔÁ¬ÐøÌØÕ÷×ö´¦Àí£¬½«Á¬ÐøµÄֵͨ¹ý¶þ·Ö²éÕÒת»»ÎªÏàÓ¦binµÄindex
¶ÔÓÚÀëÉ¢Êý¾Ý£¬binµÈͬÓÚfeatureValue.toInt
BaggedPoint£¬ÓÉÓÚrandom forestÊDZȽϵäÐ͵ÄbaggingËã·¨£¬ËùÒÔÐèÒª¶ÔѵÁ·¼¯×öbootstrap
sample
¶ø¶ÔÓÚdecision treeÊÇÌØÊâµÄµ¥¸ùrandom forest£¬ËùÒÔ²»ÐèÒª×ö³éÑù
BaggedPoint.convertToBaggedRDDWithoutSampling(treeInput)
ÆäʵֻÊÇ×ö¼òµ¥µÄ·â×°
4. DecisionTree.findBestSplits
Õâ¶Î´úÂëдµÄÓе㸴ÔÓ£¬ÓÈÆäºÍrandomForest»ìÔÓÒ»Æð
×ÜÖ®£¬¹Ø¼üÔÚ
// find best split for each node val (split: Split, stats: InformationGainStats, predict: Predict) = binsToBestSplit(aggStats, splits, featuresForNode, nodes(nodeIndex)) (nodeIndex, (split, stats, predict)) }.collectAsMap() |
¿´¿´binsToBestSplitµÄʵÏÖ£¬ÎªÁËÇåÎúÒ»µã£¬ÎÒÃÇÖ»¿´continuous feature
Ëĸö²ÎÊý:
binAggregates: DTStatsAggregator£¬ ¾ÍÊÇImpurityAggregator£¬¸ø³öÈç¹ûËã³öimpurityµÄÂß¼
splits: Array[Array[Split]], feature¶ÔÓ¦µÄsplits
featuresForNode: Option[Array[Int]],
tree node¶ÔÓ¦µÄfeature
node: Node£¬ Äĸötree node
·µ»ØÖµ:(Split, InformationGainStats, Predict)£¬
Split£¬×îÓŵÄsplit¶ÔÏ󣨰üº¬featureindexºÍsplitindex£©
InformationGainStats£¬¸Ãsplit²úÉúµÄGain¶ÔÏ󣬱íÃ÷²úÉú¶àÉÙÔöÒæ£¬¶à´ó³Ì¶È½µµÍimpurity
Predict£¬¸Ã½ÚµãµÄÔ¤²âÖµ£¬¶ÔÓÚÁ¬Ðøfeature¾ÍÊÇÆ½¾ùÖµ£¬¿´ºóÃæµÄ·ÖÎö
private def binsToBestSplit(
binAggregates: DTStatsAggregator,
splits: Array[Array[Split]],
featuresForNode: Option[Array[Int]],
node: Node): (Split, InformationGainStats, Predict) = {
// For each (feature, split), calculate the gain, and select the best (feature, split).
val (bestSplit, bestSplitStats) =
Range(0, binAggregates.metadata.numFeaturesPerNode).map { featureIndexIdx => //±éÀúÿ¸öfeature
//......È¡³öfeature¶ÔÓ¦µÄsplits
// Find best split.
val (bestFeatureSplitIndex, bestFeatureGainStats) =
Range(0, numSplits).map { case splitIdx => //±éÀúÿ¸ösplits
val leftChildStats = binAggregates.getImpurityCalculator(nodeFeatureOffset, splitIdx)
val rightChildStats = binAggregates.getImpurityCalculator(nodeFeatureOffset, numSplits)
rightChildStats.subtract(leftChildStats)
predictWithImpurity = Some(predictWithImpurity.getOrElse(
calculatePredictImpurity(leftChildStats, rightChildStats)))
val gainStats = calculateGainForSplit(leftChildStats, //Ëã³ögain£¬InformationGainStats¶ÔÏó
rightChildStats, binAggregates.metadata, predictWithImpurity.get._2)
(splitIdx, gainStats)
}.maxBy(_._2.gain) //ÕÒµ½gain×î´óµÄsplitµÄindex
(splits(featureIndex)(bestFeatureSplitIndex), bestFeatureGainStats)
}
//......Ê¡ÂÔÀëÉ¢ÌØÕ÷µÄcase
}.maxBy(_._2.gain) //ÕÒµ½gain×î´óµÄfeatureµÄsplit
(bestSplit, bestSplitStats, predictWithImpurity.get._1)
} |
Predict£¬Õâ¸öÐèÒª·ÖÎöÒ»ÏÂ
predictWithImpurity.get._1£¬predictWithImpurityÔª×éµÄµÚÒ»¸öÔªËØ
calculatePredictImpurityµÄ·µ»ØÖµÖеÄpredict
private def calculatePredictImpurity(
leftImpurityCalculator: ImpurityCalculator,
rightImpurityCalculator: ImpurityCalculator): (Predict, Double) = {
val parentNodeAgg = leftImpurityCalculator.copy
parentNodeAgg.add(rightImpurityCalculator)
val predict = calculatePredict(parentNodeAgg)
val impurity = parentNodeAgg.calculate()
(predict, impurity)
} |
private def calculatePredict(impurityCalculator: ImpurityCalculator): Predict = {
val predict = impurityCalculator.predict
val prob = impurityCalculator.prob(predict)
new Predict(predict, prob)
} |
ÕâÀïpredictºÍimpurityÓÐʲô²»Í¬£¬¿ÉÒÔ¿´³ö
impurity = ImpurityCalculator.calculate() predict = ImpurityCalculator.predict |
¶ÔÓÚÁ¬Ðøfeature£¬ÎÒÃǾͿ´VarianceµÄʵÏÖ£¬
/** * Calculate the impurity from the stored sufficient statistics. */ def calculate(): Double = Variance.calculate(stats(0), stats(1), stats(2)) |
@DeveloperApi override def calculate(count: Double, sum: Double, sumSquares: Double): Double = { if (count == 0) { return 0 } val squaredLoss = sumSquares - (sum * sum) / count squaredLoss / count } |
´ÓcalculateµÄʵÏÖ¿ÉÒÔ¿´µ½£¬impurityÇóµÄ¾ÍÊÇ·½²î, ²»ÊDZê×¼²î£¨¾ù·½²î£©
/** * Prediction which should be made based on the sufficient statistics. */ def predict: Double = if (count == 0) { 0 } else { stats(1) / count } |
¶øpredictÇóµÄ¾ÍÊÇÆ½¾ùÖµ
|