Spark
基于内存计算,提高了在大数据环境下数据处理的实时性,同时保证了高容错性和高可伸缩性,允许用户将Spark
部署在大量廉价硬件之上,形成集群。
认识Spark
Apache Spark is an open source cluster
computing system that aims to make data analytics fast
— both fast to run and fast to write. Spark是一个开源的分布式计算系统,它的目的是使得数据分析更快——写起来和运行起来都很快。
Spark 是基于内存计算的大数据并行计算框架。Spark 基于内存计算,提高了在大数据环境下数据处理的实时性,同时保证了高容错性和高可伸缩性,允许用户将Spark
部署在大量廉价硬件之上,形成集群。
Spark发展史
2009年,Spark诞生于加州大学伯克利分校AMPLab
2013年6月,Spark成为Apache孵化项目
2014年2月,Spark取代MapReduce成为Apache顶级项目
Spark生态系统
Spark拥有一套生态系统,叫做伯克利数据分析栈(BDAS)
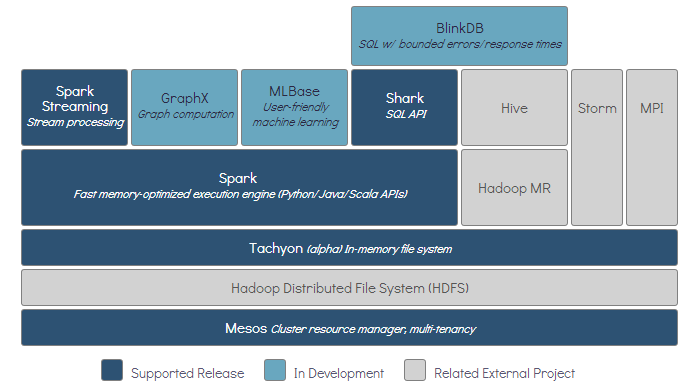
为什么比MapReduce快
Spark官网上如是说:Run programs up to 100x faster than Hadoop
MapReduce in memory, or 10x faster on disk. 那Spark究竟是为什么比MapReduce快呢?
MapReduce通常会将中间结果放到HDFS上,Spark 是基于内存计算的大数据并行计算框架,中间结果在内存中,对于迭代运算效率比较高。
MapReduce消耗了大量时间去排序,而有些场景不需要去排序,Spark可以避免不要的排序带来的开销。
Spark能够将要执行的操作做成一张有向无环图(DAG),然后进行优化。
其他优势
Spark采用事件驱动的类库AKKA启动任务,通过线程池来避免启动任务的开销。
Spark更加通用,除了具有map、reduce算子之外,还有filter、join等80多种算子。
支持的API
Scala(很好),Python(不错),Java(…)
运行模式
Local(只用于测试)
Standalone:独立模式
Spark on yarn:最有前景的模式
Spark on Mesos:官方推荐
Amazon EC2
Spark runtime
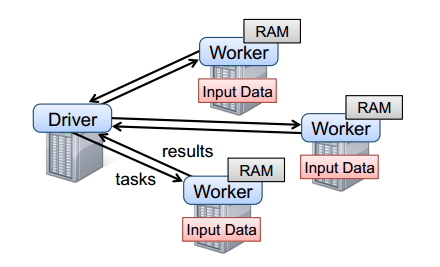
Spark运行时:用户的Driver程序启动多个Worker,Worker从文件系统中加载数据,生成新的RDD,并按照不同的分区Cache到内存中。
弹性分布式数据集RDD
RDD英文全称Resilient Distributed Dataset,即弹性分布式数据集。RDD是只读的、分区记录的集合。Spark中的一切都是基于RDD的,我们通过以下几个方面来了解它:
1、创建
1)从集合转换而来;
2)基于文件系统(本地文件、HDFS、HBase等)的输入创建;
3)从父RDD转换而来。
2、计算类型
1)Transformation(转换):延迟执行,也就是通过转换生成一个新的RDD时候并不会立即执行,只有等到Action(行动)时,才触发操作。常用操作有map、filter等。
2)Action(行动):提交Spark作业,启动计算操作,并产生最终结果(向用户程序返回或者写入文件系统)。
3、容错
Lineage:RDD含有如何从父RDD衍生出本RDD的相关信息,出错时RDD可以通过Lineage恢复。
4、内部属性
1)分区列表
2)计算每个分片的函数
3)对父RDD的一组依赖
4)对Key-Value数据类型RDD的分区器,用户可以指定分区策略和分区数
5)每个数据分区的地址列表(如HDFS上的数据块的地址)
Spark Shell
Spark自带的交互式Shell程序,方便用户进行交互式编程。进入方式:
当打开spark shell的时候SparkContext已经被初始化了,对象名为sc,直接使用即可。跟Scala解释器很像,在上面可以进行交互式操作。
接下来的内容可能需要你了解Scala语言,可以参照Scala极速入门
WordCount开胃菜
接下来我们来个实实在在的例子,作为介绍算子之前的开胃小菜。用过MapReduce的同学肯定写过Java实现的WordCount程序,如果需要排序的话还要再链接一个排序任务,逻辑不复杂、代码却也不少。我们在Spark中用Scala语言可以怎么实现呢?我们直接在Spark-Shell中操作。
scala> sc.textFile("hdfs://.../wordcount.data"). | flatMap(_ split " ").map((_, 1)).reduceByKey(_ + _). | map(x=>(x._2, x._1)).sortByKey(false).map(x=>(x._2,x._1)). | saveAsTextFile("hdfs://.../result") |
在Scala解释器中,如果输入的不是一个完整的可执行的语句,然后直接敲了回车,会出现开始的|,表示可以继续录入,直到输入一个完整的语句。也就是说我们刚刚用一行代码,搞定了WordCount
+ 排序功能。我们在后文中在对代码做一个具体的解释。对比一下吭哧吭哧写的《MapReduce统计词语出现次数》(虽然功能有一点点差异),我只能有这样的感慨:函数式编程,爽;Spark,帅!
常用算子
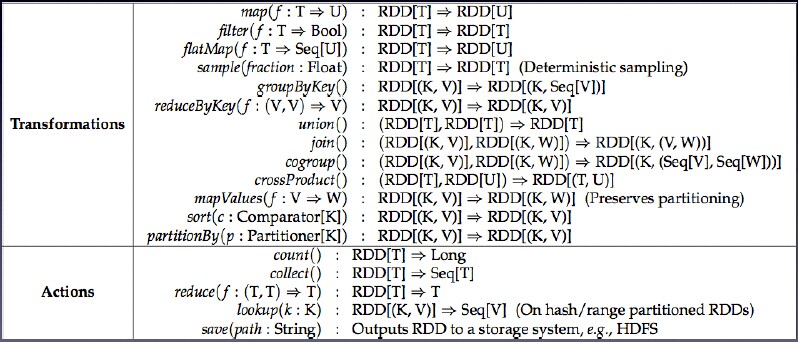
1. Spark输入:
从集合中输入:
val rdd1 = sc.parallelize(List("Java", "Scala", "Spark"))
|
从文件系统中输入:
val rdd2 = sc.textFile("hdfs://.../wordcount.data") |
2. cache
cache 将RDD 元素从磁盘缓存到内存,相当于persist(MEMORY_ONLY) 函数的功能。RDD再次使用的话,就直接从内存中读取数据。
3. map(func)
Return a new distributed dataset formed by passing
each element of the source through a function func.
将原来RDD中的每个数据项通过函数func映射为新的数据项。
val rdd2 = sc.parallelize(List(1,2,3,4)) val rdd3 = rdd2.map(_ + 2) |
rdd3从rdd2转换而来(rdd2中的每个数据项加2),rdd3中的数据项变为[3,4,5,6]。当然因为是transformations类型的算子,并不会立即执行。
4. filter(func)
Return a new dataset formed by selecting those elements
of the source on which funcreturns true.
对原RDD中的数据进行过滤,每个元素经过func函数处理,返回true的保留。
val rdd4 = rdd3.filter(_ > 4) |
rdd3中所有大于4的数据项组成了rdd4。
5. flatMap(func)
Similar to map, but each input item can be mapped to
0 or more output items (so funcshould return a Seq rather
than a single item).
与map相似,只不过每个数据项通过函数func映射成0个或者多个数据项(即func要返回一个集合),并将新生成的RDD中的元素合并到一个集合中。
6. sample(withReplacement, fraction,
seed)
Sample a fraction fraction of the data, with or without
replacement, using a given random number generator seed.
对已有的RDD进行采样,获取子集。并且可以指定是否有放回采样、采样百分比、随机种子。函数参数如下:
withReplacement = true,有放回抽样;withReplacement =false,无放回抽样。
fraction 采样随机比。
seed 采样种子,也就是一定包含在采样生成的rdd中。
7. groupByKey([numTasks])
When called on a dataset of (K, V) pairs, returns a
dataset of (K, Iterable<V>) pairs.
Note: If you are grouping in order to perform an aggregation
(such as a sum or average) over each key, using reduceByKey
or combineByKey will yield much better performance.
Note: By default, the level of parallelism in the output
depends on the number of partitions of the parent RDD.
You can pass an optional numTasks argument to set a
different number of tasks.
将含有相同key的数据合并到一个组,[numTasks]指定了分区的个数。
val rdd4 = sc.parallelize(List(("aa", 1), ("aa", 2), ("bb", 3), ("bb", 4))) val rdd5 = rdd4.groupByKey |
rdd5结果为Array[(String, Iterable[Int])] = Array((aa,CompactBuffer(1,
2)), (bb,CompactBuffer(3, 4)))
8. reduceByKey(func, [numTasks])
When called on a dataset of (K, V) pairs, returns a
dataset of (K, V) pairs where the values for each key
are aggregated using the given reduce function func,
which must be of type (V,V) => V. Like in groupByKey,
the number of reduce tasks is configurable through an
optional second argument.
将相同的key依据函数func合并。
9. union(otherDataset)
Return a new dataset that contains the union of the
elements in the source dataset and the argument.
将两个RDD合并,要求两个RDD中的数据项类型一致。
10. join(otherDataset, [numTasks])
When called on datasets of type (K, V) and (K, W),
returns a dataset of (K, (V, W)) pairs with all pairs
of elements for each key. Outer joins are supported
through leftOuterJoin,rightOuterJoin, and fullOuterJoin.
val rddtest1 = sc.parallelize(List(("James", 1), ("Wade", 2), ("Paul", 3))) val rddtest2 = sc.parallelize(List(("James", 4), ("Wade", 5))) val rddtest12 = rddtest1 join rddtest2 |
rddtest12结果为:Array[(String, (Int, Int))] = Array((James,(1,4)),
(Wade,(2,5)))
11. cogroup(otherDataset, [numTasks])
When called on datasets of type (K, V) and (K, W),
returns a dataset of (K, Iterable<V>, Iterable<W>)
tuples. This operation is also called groupWith.
对在两个RDD 中的Key-Value 类型的元素,每个RDD 相同Key 的元素分别聚合为一个集合,并且返回两个RDD
中对应Key 的元素集合的迭代器。
使用上例中rddtest1 cogroup rddtest2,结果是:
Array((Paul,(CompactBuffer(3),CompactBuffer())), (James,(CompactBuffer(1),CompactBuffer(4))),
(Wade,(CompactBuffer(2),CompactBuffer(5))))
12. sortByKey([ascending], [numTasks])
When called on a dataset of (K, V) pairs where K implements
Ordered, returns a dataset of (K, V) pairs sorted by
keys in ascending or descending order, as specified
in the boolean ascending argument.
按照key来排序,默认从小到大。如果加上参数false,则从大到小排序。
13. count()
Return the number of elements in the dataset.
返回数据项的个数。
14. collect()
Return all the elements of the dataset as an array
at the driver program. This is usually useful after
a filter or other operation that returns a sufficiently
small subset of the data.
将分布式的RDD 返回为一个单机的足够小的scala Array 数组。
15. countByKey()
Only available on RDDs of type (K, V). Returns a hashmap
of (K, Int) pairs with the count of each key.
用于key-value类型的RDD,返回每个key对应的个数。
16. lookup(key: K)
用于key-value类型的RDD,返回key对应的所有value值。
17. reduce(func)
Aggregate the elements of the dataset using a function
func (which takes two arguments and returns one). The
function should be commutative and associative so that
it can be computed correctly in parallel.
迭代遍历每一个元素,并执行函数func。
val reduceRdd = sc.parallelize(List(1,2,3,4,5)) reduceRdd.reduce(_ + _) |
计算结果为所有元素之和15。
18. saveAsTextFile(path)
Write the elements of the dataset as a text file (or
set of text files) in a given directory in the local
filesystem, HDFS or any other Hadoop-supported file
system. Spark will call toString on each element to
convert it to a line of text in the file.
将文件输出到文件系统。
注:上述算子中黑体的为Action类型的算子。
看完对算子的介绍,我们再看一下完整的程序。
if (args.length != 3) { println("Usage : java -jar code.jar dependency_jars file_location save_location") System.exit(0) } val jars = ListBuffer[String]() args(0).split(',').map(jars += _) val conf = new SparkConf() conf.setMaster("spark://host:port") .setSparkHome("your-spark-home") .setAppName("WordCount") .setJars(jars) .set("spark.executor.memory","25g") val sc = new SparkContext(conf) sc.textFile(args(1)) // 从文件系统中读取文件 .flatMap(_ split " ") // 将每一行数据,以空格为分隔符,拆分单词 .map((_, 1)) // 每个词语计数为1 .reduceByKey(_ + _) // 统计每个词语的个数 .map(x=>(x._2, x._1)) // key-value互换 .sortByKey(false) // 按照key来排序(从大到小) .map(x=>(x._2,x._1)) // key-value互换 .saveAsTextFile(args(2)) // 将结果输出到文件系统中 |
总结
本文简单介绍了Spark中的一些概念,并结合了一些简单的例子。内容写的比较浅显,随着笔者的深入研究,也会在后续博客中对各部分内容做更深入的阐述。希望本篇能对看到的人有所帮助。
|